Self-Driving Cars are Leveling Up in Autonomy
A look at the self-driving carmakers’ road ahead.
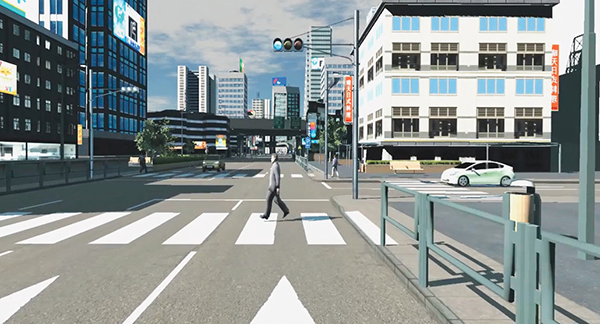
With ANSYS’s VREXPERIENCE software, car developers can use VR-enabled simulation to see how the vehicle’s navigation reacts to different weather, traffic and road conditions. Images courtesy of ANSYS.
Latest News
May 1, 2019
Who is a better driver: the human or the artificial intelligence (AI)? That may depend on what you mean by “better.” For many AI systems, the hallmark of good driving is to be able to detect the lane ahead and place itself at the dead center of that lane. In general, this may be desirable, but there are situations where this is ill-advised.
“When you notice a pothole or a damaged road ahead, you nudge your steering wheel ever so slightly so you can keep the obstacle between your two wheels as you drive past it,” points out Chris Hoyle, technical director for rFpro, a drive simulation software developer. “You want your AI system to be able to recognize these damaged roads and potholes and react in the same way.”
The replication of human driving behavior—even the ill-advised swerves and lane changes of a tired, irrational driver—is important for another reason. If the virtual drivers in the simulated traffic abide by all the traffic rules and seem to know all the speed limits and lane changes with ample warnings ahead, then they’re not behaving like typical human drivers. An autonomous navigation algorithm developed under these ideal conditions may not know how to react to the irrational, imperfect navigations of real humans on the road.
“It’s not enough to come up with a nicely random simulation; you have to come up with hundreds or thousands of driving styles,” says Hoyle.
It’s neither safe nor practical to put unproven autonomous vehicles on the public road for testing. Therefore, most developers would invariably rely on simulation to make sure their vehicles can make the right decision, even in the highly unusual “edge” scenarios. The type of sophisticated simulation and training now possible suggest that moving from the current level of autonomy (Level 2+) to conditional and full autonomy (Level 3, 4, 5) is not very far off.
What Level Are We At?
The five levels of autonomy as defined by the Society of Automotive Engineers (SAE) set up clear expectations for autonomous car developers (see chart below). The SAE’s J3016 Levels of Automated Driving Chart has also been adopted by the U.S. Department of Transportation (DOT) as a policy-guiding document. It goes from Level 0: No Autonomy to Level 5: Full Autonomy.
“There’s Level 2 technology in the cars commercially available today,” notes Sandeep Sovani, director of global automotive industry, ANSYS. “These are advanced driver-assistance systems. In other words, the driver still has to keep full control of the vehicle; the systems merely help the driver.
Although the passenger cars are still between Level 2 and 3, the transportation industry has already surpassed them, according to Jonathan Dutton, marketing director, transportation and mobility industry, Dassault Systèmes.
“Some transportation companies are already testing and deploying small buses in geofenced regions in smart cities,” says Dutton. “Today, there’s generally a human operator on the bus keeping an eye on things. But these vehicles are already operating in the fully autonomous mode.”
For example, with its headquarters in Lyon, France, and R&D facilities in Paris, NAVYA offers autonomous shuttle (Autonom Shuttle) and autonomous cabs (Autonom Cab). Some of its vehicles are in service in Singapore, Perth, Australia); Christchurch Airport in New Zealand; and Curtin University in Australia. Such conditional autonomy with the condition being the restricted zone will fall under Level 4 as defined by the SAE.
The Pesky Level
For Level 0 to Level 2, the human driver is fully or largely responsible for road monitoring and making appropriate decisions. For Level 3 to Level 5, the system plays an increasingly larger role, executing dynamic navigating tasks and reacting to events. Therefore, the leap from Level 2 to 3 marks a significant breakthrough.
“Some companies are talking about skipping Level 3 and going straight to Level 4 or 5,” says Sovani. “In Level 3, the human driver needs to take control of the vehicle at a moment’s notice if something is amiss. But humans just don’t seem capable of this kind of timely reaction.”
In an article published November 3, 2017, Car and Driver magazine revealed, “Toyota is uneasy about the handoff between automated systems and drivers.” In its online declaration titled “Looking Further,” American carmaker Ford said, “[By] 2021 … [the] vehicle will operate without a steering wheel, gas pedal, or brake pedal within geofenced areas as part of a ride-sharing or ride-hailing experience. By doing this, the vehicle will be classified as a SAE Level 4 capable-vehicle.” However, last month Ford CEO Jim Hackett publicly acknowledged that the vehicle’s application will be limited because autonomous driving is more complex than the industry anticipated.
Part of the difficulty with designing a Level 3 car is human nature itself. Can a human be relaxed and alert at the same time? A driver may physically and mentally be capable of taking control of the car, but he or she may be highly absorbed in a game, a movie or a chat, preventing the takeover from occurring in a timely fashion.
GPU-accelerated AI Training
Focusing on GPU-driven autonomous car development, graphics processing unit (GPU) maker NVIDIA has developed an autonomous vehicle platform. Its DRIVE AP2X Level 2+ AutoPilot uses technology from the higher levels of autonomy.
“DRIVE AP2X is a Level 2+ automated driving system. The driver is still responsible and must monitor the road, but we’re also incorporating surround sensors and AI software running on deep neural networks to protect the driver and the passengers in the car,” says Danny Shapiro, senior director of automotive, NVIDIA. “The technology also includes driver monitoring, and can issue alerts or take action if the driver is distracted or drowsy.”
At the NVIDIA GPU Technology Conference (GTC) in March, NVIDIA announced its autonomous car simulation platform NVIDIA DRIVE Constellation is now available. It is a data center solution comprised of two side-by-side servers. The DRIVE Constellation Simulator server uses NVIDIA GPUs running DRIVE Sim software to generate the sensor output from the virtual car driving in a virtual world. The DRIVE Constellation Vehicle server contains the DRIVE AGX Pegasus AI car computer, which processes the simulated sensor data. The driving decisions from DRIVE Constellation Vehicle are fed back into DRIVE Constellation Simulator, enabling hardware-in-the-loop testing.
DRIVE Constellation is an open platform, and can incorporate many third-party world models, vehicle models, sensor models and traffic models. Recently, the Toyota Research Institute-Advanced Development, the R&D arm of the Japanese carmaker, has announced it will use NVIDIA DRIVE Constellation to test and validate its autonomous vehicle systems.
Role of Simulation
Modern passenger cars benefit from the cumulative experience of an industry that has been crash-testing for decades. To achieve a comparable type of reliability, the connected autonomous cars must be road-tested for millions of miles. This is something that is highly challenging to do in the congested physical world, but could be accomplished in a much shorter time in the virtual world.
“The reason the current generation cars perform quite well in crash situations is because they have gone through many crashing tests in the physical environment,” says Dutton.
In January of this year, Dassault Systèmes struck a strategic partnership with Cognata, which provides an autonomous vehicle simulation suite. Announcing the deal, Dassault Systèmes wrote: “By incorporating the Cognata simulation suite into [Dassault’s] 3DEXPERIENCE platform and leveraging CATIA … the two companies deliver a one-stop-shop, outstanding environment to engineers for accelerated autonomous vehicle design, engineering, simulation and program management.”
In 2017, Siemens PLM Software acquired TASS, an autonomous driving simulation software developer. As a result, TASS’ PreScan software is now part of the company’s portfolio.
PreScan is a physics-based simulation platform that is used in the automotive industry for development of advanced driver assistance systems (ADAS) that are based on sensor technologies such as radar, laser/lidar, camera and GPS, according to the company. PreScan also can work with accident information, such as road traffic accident data from the German In-Depth Accident Study (GIDAS) project.
“In PreScan, you can have a cyclist jump out in front of your car; you can change the weather from rainy to snowy to icy; and you can add more complexity to your driving scenarios,” explains Andrew Macleod, director of automotive marketing, Mentor, Siemens PLM Software.
The Role of VR
In May 2018, ANSYS acquired OPTIS, which develops optical sensor and closed-loop, real-time simulation software for car makers. As a result, ANSYS added VREXPERIENCE, an autonomous driving simulator, to its offerings.
“The VREXPERIENCE software has a suite of virtual sensors mounted on the virtual car, so as you drive your car through the simulated traffic, these sensors are capturing the road information. This represents what a real car would see when they drive this road,” explains Sovani.
rFpro also accommodates the use of VR to allow developers to test their AI with real human road users in the loop. “The most cost-effective setup is for you to sit at a desk, with a VR headset providing the full 3D world, with your feet on a couple of pedals, with a steering wheel in front of you,” says Hoyle. This provides a more realistic testing environment, because a 2D flat screen doesn’t offer the same peripheral vision that a driver relies on for navigation.
“The VR hardware has just improved enough for this application,” says Hoyle. “Earlier versions are not suitable due to weight and heat. Remember, you have to strap this device to your forehead, so if it’s too heavy or too hot, it wouldn’t be pleasant. The low resolution and latency in the earlier units gave users motion sickness. In VR, you can still test your AI in demanding real-world situations, surrounded by real road users, but without risk of injury.”
Stay in the Safe Zone
Last October, Volvo announced it will use NVIDIA DRIVE AGX Xavier platform for its new core computer for assisted driving that will go into every next-generation Volvo. At CES 2019, suppliers Continental and ZF announced their production plans for Level 2+ systems built on NVIDIA DRIVE, with production starting as early as 2020. Mercedes-Benz also announced that its next-generation centralized computing architecture for AI in the cockpit and AI for self-driving will use NVIDIA DRIVE technology.
At GTC, NVIDIA unveiled its safety driving policy called the Safety Force Field (SFF). which is integrated into the NVIDIA DRIVE technology stack. “SFF is a robust driving policy that analyzes and predicts the vehicle’s environment. It determines a set of acceptable actions to protect the vehicle, as well as others on the road. These actions won’t create, escalate or contribute to an unsafe situation, and include the measures necessary to mitigate harmful scenarios,” the company explains.
Likely Scenarios for the Near Future
Before fully autonomous private vehicles appear on the road, you may begin to see highly autonomous robo taxis, with a safety driver monitoring it remotely, Sovani envisions.
“Today, the taxis have human safety drivers. But in the next few years, you’ll likely see the safety driver become a remote driver. In other words, for each vehicle, there may be someone at a central control station, remotely monitoring the camera’s views,” according to Sovani’s vision. “It will be a few years before fully automated robo taxis are commercially deployed in large numbers, as all the imaginable incidents that can occur in a driving session are too numerous for any software to account for.”
On-demand mobility service providers like Uber and Lyft are interested in Level 4 and 5 autonomy. So are densely populated cities with mass transit challenges. “In that setup, I can’t foresee human drivers and autonomous cars sharing the road. The mixture is not safe. What we need are vehicles that can talk to one another, and also talk to the infrastructure, such as traffic lights. But a lot of investment has to happen before we get there,” says Macleod.
Looking ahead to Level 4 and 5, Macleod believes manufacturers need to make the autonomous vehicles highly customizable. A single model may not be suitable for all cities. “It would have to be batch manufacturing that’s configurable,” he reasons.
“Level 4 and Level 5 vehicles will have more sensors, higher resolution sensors and will require an AI supercomputer capable of processing all that data through many deep neural networks,” notes Shapiro. “The NVIDIA DRIVE AGX Pegasus is the platform that many robo taxi and autonomous delivery companies are using as it is capable of processing 320 trillion operations per second.”
Suppose autonomous trains, buses and robo taxis become common. Would people still want to own private cars, autonomous or otherwise? Dutton has serious doubts. “You’ll always need big buses and trains to commute to work from the suburbs; and in places where you still need to go to your destination from the bus station or train terminal, an add-on transportation service can take care of it. If transportation becomes that easy, why would you want to own a car?”
More Ansys Coverage
More Dassault Systemes Coverage
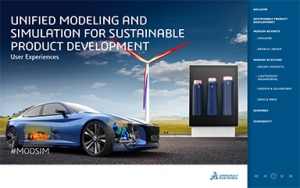
More NVIDIA Coverage
More Siemens Digital Industries Software Coverage
Subscribe to our FREE magazine,
FREE email newsletters or both!Latest News
About the Author

Kenneth Wong is Digital Engineering’s resident blogger and senior editor. Email him at [email protected] or share your thoughts on this article at digitaleng.news/facebook.
Follow DE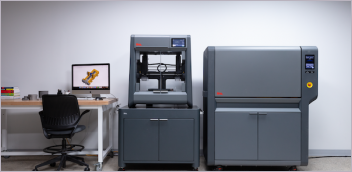
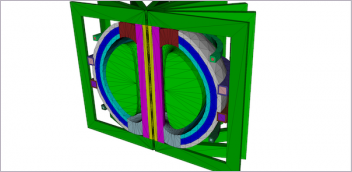
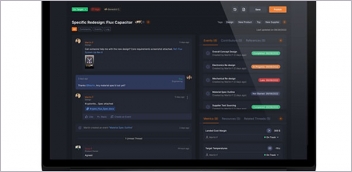
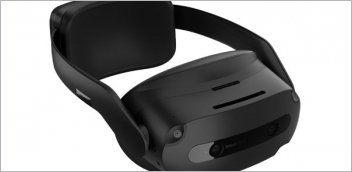