Aerospace Digital Twins Gain Altitude
As digital twin capabilities expand, aerospace companies can gain more insight into the entire aircraft lifecycle.

Shown here is a full 3D digital model of a Trent jet engine. The propulsion system consists of 30,000 parts, which are modeled as hundreds of individual assemblies. Image courtesy of Rolls-Royce.
Latest News
March 16, 2022
The aerospace industry and its supply chain partners have embraced the promise of the digital twin. The sector’s leaders consider the shift to the virtual world to be an essential enabler of the industry’s digital transformation. The key takeaway, however, is not aerospace’s adoption of digital twin, but rather how and when engineering teams choose to apply the technology.
Unlike many segments of the market in which companies just dip their toes in the water by implementing small, isolated digital twin test initiatives, the aerospace industry is using the technology throughout the entire product lifecycle, from design and manufacturing to operations and maintenance.
That said, aircraft manufacturers are still tailoring the technology’s use to conform to its current development practices. This translates into an approach in which aircraft companies begin using digital twin with smaller, simpler design elements and work up to more complex systems.
“We are incrementally advancing digital twin technology to move from individual components to systems, which allows us to scale the capability to ‘see’ and predict more of the platform,” says Don Farr, senior technical fellow, digital transformation with Boeing Research and Technology.
The idea behind this approach is that as digital twin capabilities continue to expand, digital threads will lengthen, and digital twins will increase in resolution and give aerospace companies more insight into all phases of the aircraft’s lifecycle. This, in turn, allows designers to improve safety, reliability and operational efficiencies.
Design’s Virtual Crystal Ball
The digital twin’s first impact on aerospace product development and management occurs during the design process. Here, the virtual technology provides unprecedented visibility and flexibility, which allows aerospace companies and their suppliers to more rapidly, cost-effectively determine whether different technologies and architectures can deliver what the customer needs (Fig. 1).
“Digital twins are being used to develop a robust understanding of the product, its performance, and the associated production processes,” says Dale Tutt, vice president of industry strategy for Siemens Digital Industries Software. “This understanding allows business and engineering leaders to make more informed decisions as they develop and support products.”
Aircraft and component manufacturers now bring this insight to better predict, detect and address quality trends, reduce engineering variability, shrink product development time, optimize maintenance and engineering, manage inventory and reduce storage costs.
Currently, aerospace companies place great emphasis on using the digital twin to confirm that aircraft and subsystem designs will perform correctly and that they meet all stakeholder requirements.
“The digital transformation goal for digital twins is to conduct as much of the validation and verification in the virtual domain as possible,” says Farr. “For example, a platform drop test—when we physically drop a platform from a specific height and verify the design—has limitations in the physical domain. A high-fidelity virtual representation of the platform, however, can exercise many more variables [for example, drop angles] than what can be accomplished in the physical space. The benefit of this is that the digital twin and physical platform work together to provide input and calibration during testing.”
Better Visibility, Better Maintenance
Digital twins also have influence beyond the drawing board, into the field, which improves aircraft maintenance regimens and extends the plane’s operating life. The approach used represents a marked departure from traditional practices.
Previously, engineers would typically model and evaluate key aircraft aspects by comparing them with reference operational cycles, and from that, they would determine if a given airframe met the required safety standards. This would sometimes be enhanced with periodic in-service inspections, but broadly speaking, the lifecycle cost was fixed by an estimate of a safe average behavior.
The new approach, however, calls for safety determinations to be made based on operational data extracted from sensors that monitor real-world conditions. This increased reliance on sensor data is driven in part by advances in digital twin capabilities.
“Real sensor data has always been used from tests or operation of the product to correlate the modeling and simulation results with real-world data, but it was often a laborious process,” says Tutt. “With current digital twin technology, greater sensor data availability and more powerful data analytics/machine learning, leading companies are accelerating the pace at which they can update their models and simulations.”
These new capabilities allow engineers using digital twins of operational components, systems or aircraft to report the asset’s current condition and move from a reliability-based maintenance program to a condition-based maintenance approach. This results in a more efficient maintenance schedule.
For example, instead of performing maintenance at recommended intervals, digital twins monitor the health and efficiency of components and allow maintainers to schedule maintenance as required. This can be further optimized when the company includes historic operational environment data for each aircraft.
“We’re including aircraft operational data in our considerations at Boeing,” says Darren Macer, senior technical fellow, predictive maintenance and airplane health management at Boeing Global Services. “Once an aircraft enters into the operational phase of its lifecycle, Boeing’s analytics teams take the digital twin for components or systems of interest on that aircraft and maintain those using data related to their physical counterparts.”
As Macer explains, Boeing collects flight sensor data, maintenance records, and operational and environmental data. All data is stored, correlated, tagged and enhanced.
“Because our ability to process data has expanded, we can now connect all the known information about an aircraft platform, and not just connect it, but dynamically update and maintain it,” Macer adds. “As we continue to scale the resolution and coverage of these operational digital twins, our capability will expand to cover more of the complete aircraft.”
Taking Maintenance to the Next Level
Advances in digital twin and sensor connectivity technologies, combined with artificial intelligence and powerful analytics, have laid the groundwork for the next generation of aerospace maintenance practices (Fig. 2).
“Companies are expanding predictive health monitoring using advanced analytics with digital twins,” says Tutt. “Combining real-time sensor data with the digital twin enables companies to detect trends of component or system performance, and then provide alerts when preventative action needs to be taken. This results in safer operations and reduced maintenance costs.”
One such use case might involve engines used on commercial aircraft. These propulsion systems are fitted with sensors that feed pressure, temperature, speed and vibration measurements from critical locations to a health and condition monitoring unit that periodically returns the information to a digital twin.
The digital twin takes this sensor data and fuses it with external information and records, such as weather conditions, which enables maintenance teams to analyze data using predictive algorithms. These forecast how much of the product’s life has been consumed and review records to see if any anomalies have been flagged by the control system, such as an unexpected vibration. The maintenance team then uses the digital twin models to evaluate possible causes and recommends corrective action.
For example, if the maintenance team sees a vibration signature linked harmonically to a shaft speed, they know from experience that the vibration could be a sign of a damaged bearing.
Maintenance teams also can do more complex checks. They can relate the engine acceleration and speed to the flight phase. If teams see a sudden small change in speed during takeoff, it could be a sign that a foreign object has been sucked into the intake. They would then instruct an engine check by borescope to look for signs of damage in a particular area, depending on the shaft affected.
Fig. 3: Shown here is a full 3D digital model of a Trent jet engine. The propulsion system consists of 30,000 parts, which are modeled as hundreds of individual assemblies. Image courtesy of Rolls-Royce.
“We aggregate these records over the engine’s operating life to proactively manage product life and time between overhauls,” says Chris Young, group chief engineer at Rolls-Royce. “This gives the customer more uptime and lower through-life costs. So, we can work out how each asset is being used and from that information plan a bespoke set of on-wing services and eventual repair and overhaul tailored to what the engine has seen.”
With these predictive practices, maintenance teams also can model how well an asset has been used, and unlock numerous options. For example, they could model how well an operator is making use of fuel, evaluating ways to optimize routes and pilot training to reduce fuel costs, or the maintenance team could provide an end user with reports to correctly assess carbon and other emissions per flight.
Within this context, predictive maintenance is still in its early days. Even so, it is gaining real traction among aerospace companies.
“Aerospace companies are developing smart digital twins to utilize sensor data and analytics to develop predictive models that simulate and predict future conditions to support MRO [maintenance, repair and operations],” says Farr. “Already, digital twin technology, bolstered by AI and machine learning tools, enable us to offer more predictive maintenance. These practices go beyond recommendations and enable streamlined maintenance planning to enhance operational efficiency—from predicting and identifying parts to replace to automatically updating maintenance task cards.”
Hurdles Remain
While aerospace companies have made great strides using digital twin technology, there are still obstacles to overcome. Most have to do with the daunting scale of applications.
“Current technology can handle some isolated aspects of the modeling and simulation for specific subsystems or focused combinations of environmental and vehicle interaction dynamics,” says Mike Geyer, Omniverse manufacturing industry lead at NVIDIA. “The challenges arise in simulating and evaluating complex systems of systems.”
The problem becomes scaling up the technology’s capacity to cope with the number of records in a useful digital twin, as well as finding the IT storage required to accommodate the data. Digital twin technology is pushed to the limit when engineers must index the massive numbers of models, records and decisions that define the twin (Fig. 3).
For every turbine aerofoil that Rolls-Royce designs, the company assigns a part number, which acts as an overall identifier for hundreds of designs and manufacturing data objects that describe what it looks like, how it works and how it is manufactured and inspected.
Under every part number, Rolls-Royce stores numerous supply chain records, each identified by a serial number. These records include orders and manufacturing records and associated processing and inspection data, such as coordinate measurements, X-rays and digital scans.
Each serial number can be linked to gigabytes of information. It is simply impractical to store all of that raw data for every part.
To remedy the situation, Rolls-Royce is working on ways to reduce the data to a surrogate twin that can be used to simulate how each individual part and assembly behaves through decades of operational life.
“The race now is to make it practicable to store the information for every part and assembly on a production basis—thousands in a jet engine and potentially millions in something like a small modular reactor,” says Young. “This needs to be addressed by scalable data storage, which means finding an affordable yet secure cloud solution to augment the current on-premises stores, and also understanding how to pipeline raw data and reduce it into useful datasets and models that can be affordably stored and made accessible throughout the lifecycle.”
The level of complexity is further heightened by nagging problems present wherever data is collected and digital threads are implemented.
For example, according to David Ziegler, vice president of industry aerospace and defense at Dassault Systèmes, one problem arises when the twin tries to access data that may belong to the operator, not the original equipment manufacturer.
Likewise, getting the latest as-running condition of an asset operating in the field using Internet of Things sensors can also be problematic.
“The ability to have end-to-end traceability—digital thread—from an operating asset in the field as to how it was manufactured and/or designed, and the requirements that drove the design, is often challenging because most of the data is still in its own silo, which are seldom connected,” says Suresh Iyer, vice president of MRO solutions at Aras.
Digital Twin’s Evolving Footprint
By pushing digital twin technology to its limits, the aerospace industry has assumed a major role in defining what these virtual systems will look like tomorrow. As aircraft makers and their supply chain partners develop smart digital twins that more efficiently use sensor data and analytics, they advance the twin’s horizon from performing verification and validation functions to enabling more pervasive use of predictive maintenance in support of MRO.
These major shifts reflect underlying changes as well.
“Digital twin’s evolution has driven us to move from a culture where parts are drawn to instruct factories to meet key dimensional requirements set from datums, to where parts are fully modelled as solid objects in three dimensions and described by a computer-aided manufacturing process,” says Young. “In turn, this is forcing the industry to move to a complete PLM [product lifecycle management] approach, to a place where we model the design in a suite of tools interlinked with the PLM configuration and design data.”
This means that the simulation suite must fit within the end-to-end process of design and approval. Compatibility between CAD modeling standards and analysis techniques, as well as among systems of different enterprises, such as between the engine provider and the airframer, are equally critical.
More Aras Coverage
More Dassault Systemes Coverage
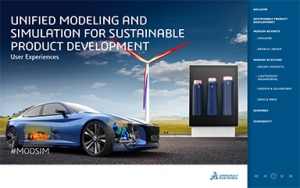
More NVIDIA Coverage
Subscribe to our FREE magazine,
FREE email newsletters or both!Latest News
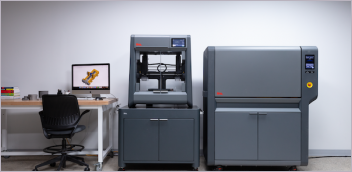
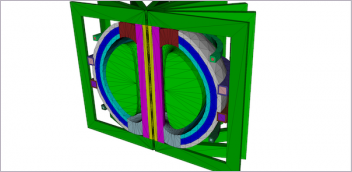
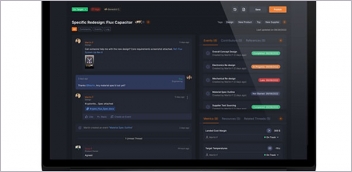
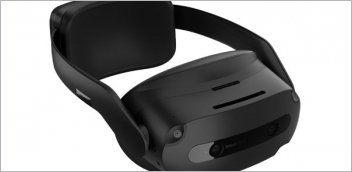