
Achates Power develops opposed piston engines, which it says are 50% more efficient than current gasoline engines. This proof of concept was created to show how the engine could be adapted to fit under the hood of a Ford F150 truck. Image courtesy of Achates Power.
April 28, 2023
Wind turbines have proven to be complicated to assemble with traditional construction cranes. As a result, multinational crane manufacturer Liftra decided it needed a new approach, and asked its engineering team to design a more compact crane that would literally climb up the tower as assembly progresses. The goal was to have a crane that would occupy less ground space and only require one truck for transportation to the site. At the same time, they had to ensure tower components could withstand the climbing forces, including the weight on the tower and flanges as well as the grip strength.
To assess the impact of crane attachment and ascent on the assembly of wind turbines, Liftra engineers conducted static simulations of the loads involved using finite element structural simulations. Linear and nonlinear material models were used to perform elastic/plastic analysis of the towers and determine the extent of any permanent deformation caused by the crane.
The optimal simulation configuration involved using a combination of Ansys Cloud, Ansys Elastic Licensing and Liftra’s software licenses. The engineering team took advantage of licenses from offices in Spain and Denmark, borrowing them as needed to keep the simulations moving.
The linear material model was run using Ansys Cloud on an HC Virtual Machine powered by 44 Intel Xeon Platinum 8168 processor cores. The model incorporated more than 1.8 million nodes, including more than 100 nonlinear contacts. The simulation was completed within an hour on Ansys Cloud, whereas it took approximately 5 hours on company workstations, a five-times acceleration in processing time.
A cost analysis determined it was better for Liftra to use its licenses on cloud time rather than making improvements to an on-premise cluster. The company benefited from reduced maintenance expenses and IT compensation for managing the cluster, and they also got by with using fewer licenses.
These kinds of results are no longer uncommon. Manufacturers are becoming more willing to add cloud-based simulation to their toolset. Finite element analysis, computational fluid dynamics (CFD) and other primary simulation types are being adapted for cloud, and more companies are taking notice. Recent arrivals to the market include Siemens Simcenter and Ansys Gateway, both powered by AWS.
Simultaneous Simulations
A recent collaborative research project in Germany successfully exploited cloud computing and artificial intelligence to develop a strategy for “net zero” hydrogen storage and transportation. Cloud services company Kaleidosim and CFD provider MultiFluidX created a solver that combines CFD and machine learning. A system running OpenFOAM on Kaleidosim’s cloud platform allowed for simultaneous simulations. Comparing the machine-learning-enhanced results with a more traditional approach confirmed close correlation with existing CFD data. The research team said the cloud/AI approach was easy to set up, and gave results in seconds.
SilTerra is a global semiconductor foundry based in Malaysia. For a next-generation series of new micro-electromechanical systems/complementary metal-oxide semiconductor devices, SilTerra used OnScale Cloud Simulation from Ansys to “digitally prototype” performance, quality and yield early in the product development process.
Cloud-based simulation services manager Kaleidosim recently conducted a test with computational fluid dynamics (CFD) provider MultiFluidX, testing a solver that combines CFD and machine learning. Close correlation was confirmed with traditional approaches, with a significant reduction in compute time. Image courtesy of Kaleidosim.
One client needed to mimic ultrasound device performance underwater. SilTerra wanted to generate a product design kit—a set of models—to allow them to start using simulation as a tool for improving new designs and decreasing time to market. Typical turnaround for a project like this would typically be 7 months. By using OnScale on AWS to simulate the project, SilTerra says it was able to turn around the ultrasonic cell design in 3 days. It credits OnScale’s flexible licensing, fast solvers and the ability to run multiple simulations simultaneously for a 60% total cost savings, with similar reductions in cycle time and engineering resources.
“OnScale helps us to demonstrate our capabilities faster by greatly reducing the cycle time for prototyping,” says Arjun K. Kantimahanti, a senior vice president at SilTerra. “Key insights into the end product enable us to tweak the design and performance to achieve optimized products faster.”
No More Shortcuts
A 2020 study by Ansys found that engineers were reducing the fidelity and detail of their models more than 50% of the time to meet required turnaround times. Such findings, along with the falling costs of using cloud resources and the speed of cloud-based simulations, contributed to the company’s embrace of cloud simulation as the way forward. The gain in compute power from cloud computing allows engineers to run larger, more complex simulations with greater fidelity.
“This combination of scalability and flexibility enables clients to do more simulations faster without being constrained by the limits of fixed on-premises resources,” notes Rod Mach, president of TotalCAE, which specializes in managing compute resources for engineering companies. TotalCAE started 20 years ago as a resource for high-performance computing (HPC) clusters, but now is seeing a majority of its work in helping engineering teams get the most from cloud services.
“The compute resources are globally available, which makes it easier to collaborate or to monitor simulation jobs anytime, anywhere,” Mach says. “Small businesses have access to the exact same HPC resources as the largest companies to level the competitive playing field.”
Considerations for the Shift
As cloud-based simulation becomes more popular, somebody has to optimize processes originally meant for on-premise use. Most engineering groups are using services such as TotalCAE or Rescale, or relying on support from the simulation vendor. Achates Power chose the managing partner approach by asking TotalCAE to provide them a turnkey cloud-based simulation platform.
Achates Power develops Opposed Piston Engines, a next-generation internal combustion engine that increases fuel efficiency, reduces greenhouse emissions and costs less than conventional engines. Achates needed additional compute capacity for a short-term high-priority project. Adding new computers to the existing cluster would take too long, so the company turned to TotalCAE.
“TotalCAE made it simple to incorporate burst-to-cloud with our existing TotalCAE cluster,” says Rodrigo Zermeño, a senior engineer at Achates Power. “We just pointed our browser to a new URL and we were able to keep using the same intuitive web interface to run our applications on AWS.”
Some teams have resources to extend to the cloud—or replace in-house compute resources with cloud computing. For them, here are a few key considerations when rewriting FEA or CFD protocols for cloud computing:
- Data Preparation: Optimize data for cloud storage by compressing and eliminating duplicates, while maintaining sufficient data integrity for simulations.
- Parallel Computing: Break down the simulation into multiple sub-tasks and distribute them across multiple machines to speed up simulations.
- Scalability: Create simulation protocols that can scale in processing power, storage and data bandwidth—ensuring computational time is reduced.
- API Integration: Use application programming interfaces to enhance data integration, preprocessing and post-processing of FEA simulations.
More Ansys Coverage
Subscribe to our FREE magazine,
FREE email newsletters or both!About the Author

Randall S. Newton is principal analyst at Consilia Vektor, covering engineering technology. He has been part of the computer graphics industry in a variety of roles since 1985.
Follow DE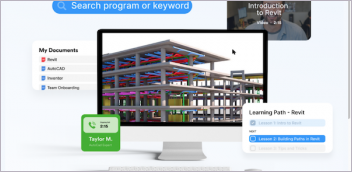
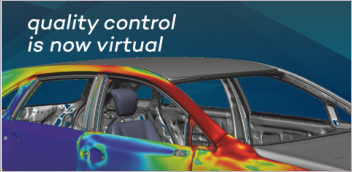
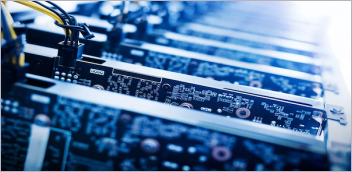
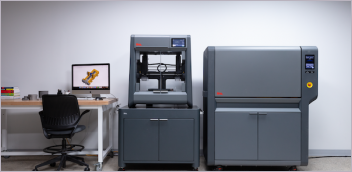