PLM: Obsolete Technology or Work in Progress?
As data usage expands, PLM platforms must evolve to support new types of data and connectivity across disciplines.

For PLM systems to remain relevant, they must evolve from stand-alone applications to multi-engineering domain suites of applications. Here, a PLM platform enables the left screen to depict the propeller’s structural information and the right screen to show stress analysis of the same component. Image courtesy of Dassault Systèmes.
November 1, 2021
Dramatic changes are afoot with product lifecycle management (PLM) technology, with engineers, manufacturers and industry watchers raising a number of prickly questions. For instance: Are PLM platforms still relevant? Can software developers adapt these platforms to meet the latest wave of demands (Fig. 1)? Or are PLM platforms fated to fade away like so many other technologies that have preceded it?
To answer these questions, it is imperative to understand what these platforms were originally designed to do and examine how well PLM architecture meets or fails to meet current challenges facing the technology.
Only then can you determine whether PLM will continue to serve a purpose in the engineer’s toolbox or simply disappear from the data management ecosystem.
A Product of Their Time
Traditional PLM systems are products of a time when files were the predominant medium for storing product information and when teams worked almost exclusively in the same physical location.
These platforms were originally written as proprietary, out-of-the-box software aimed at reducing the time spent managing files and development processes. Until recently, PLM software has focused almost solely on the work of mechanical engineering teams. As a result, they were designed to handle CAD drawings and technical engineering data, often in a fashion not user friendly to those working in other engineering domains.
All these factors make for a rigid architecture, suited to handle only a small subset of the data now used to design and manufacture new products. The stand-alone nature of these proprietary platforms makes modifications and upgrades difficult and often precludes interaction with other types of software. These limitations have essentially placed a cap on the value the traditional PLM systems can deliver.
“The main challenge today is that savvy businesses are quickly realizing there’s an opportunity to start using that data in ways that extend beyond the development process and create new value for the business and their customers,” says Loren Welch, director of design and manufacturing strategy at Autodesk.
“Unfortunately, traditional PLM systems weren’t built to work this way and do not yield these kinds of insights,” Welch adds. “As a result, massive amounts of product development data are literally just sitting there on servers doing little more than taking up space.”
Coming to terms with these realities, it’s become clear that PLM systems must grow to manage more data types, in much greater quantities plus support data connectivity across all relevant domains and disciplines.
This means integrating all engineering data—including mechanical, electrical, electronics, software and systems engineering—to create a real-time holistic view of the asset in question. This is easier said than done.
“All these changes put pressure on traditional PLM architectures,” says Bill Lewis, director of marketing for Teamcenter, Siemens Digital Industries Software. “If a traditional architecture can’t evolve to accommodate all of these things, they won’t be able to keep up with what customers are demanding from the products that they buy, and they won’t be able to support the new business models that original equipment manufacturers (OEMs) are looking to capitalize on.”
Problems From the Start
Pressure points provide a clear view of where and how traditional PLM architectures fail to meet the demands of today’s product development and manufacturing teams. One such pressure point is front-end design data.
Products have become more sophisticated as product development teams increasingly use the latest generation of design tools, such as 3D CAD and advanced modeling and simulation software. This in turn has increased the data that must be tracked, managed and scaled to uncover insights that increase productivity.
Further complicating the data management process, CAD software vendors have built traditional PLM systems to manage their proprietary CAD data. Most businesses, however, use multiple authoring applications, from various software vendors. These include electronic design automation, modeling and simulation, computer-aided manufacturing and application development tools. Accommodating all these integrations and file compatibilities has become very difficult, if not impossible, for proprietary on-premises PLM systems.
A solution emerging in modern PLM systems, especially those based on the cloud systems, is the adoption of new data storage strategies.
“Traditional PLM systems were file based,” says Ramesh Velaga, worldwide ENOVIA industry process expert at Dassault Systèmes. “To support the needs of new product development, newer PLM systems are eliminating files and moving to data-based representations.”
This allows businesses to tap a single-source-of-truth dataset, whatever the current product design is, as the source of data they analyze. These systems eliminate delays, loss of detailed data and headaches that come with file switching.
Big Data Equals Big Problems
Another pressure point of interest can be found in the infusion of data from Internet of Things (IoT) devices—also known as Big Data.
Users that implement digital threads and digital twins now ask PLM systems to incorporate IoT data to create a complete picture of an asset, extending through the product’s operating life. This promises to open the door for engineers and manufacturers to evaluate the asset’s performance, improve existing designs and manufacturing strategies and create new products (Fig. 2).
“The advent of the IoT and other data collection technologies has created a new avenue that clients can use to understand the performance of their products in real time,” says Velaga. “This data helps them to build reliable and fault-tolerant products.”
The catch is that the quantity and diversity of the data in question is unlike anything PLM has ever seen.
“The volume of data that PLM systems are now supposed to handle can be staggering,” says Matthew Thomas, managing director and North America engineering lead at Accenture. “For example, we created a digital twin for an aerospace company of an in-production component that had millions upon millions of data points. PLM systems are just not architected to handle this type of data in an effective way.”
A growing consensus is emerging that contends that virtually nobody wants, or has the capacity in their PLM systems, to store and manage the data on-site in the same way they manage design data. It is simply too much data for on-premises systems to ingest.
Software Enters the Picture
Much like the infusion of IoT device data, the increasing importance of software development data is forcing PLM users to take a hard look at the fundamental nature of the next generation of products and how it impacts the effectiveness of traditional PLM architectures.
“In the past, a bill of materials for a product was often driven by computer-aided design,” says Thomas. “Today, innovation is more likely going to come from the software rather than electronics or hardware designs. This is a whole different way of thinking about a product.”
Confronted with these facts, PLM developers now acknowledge the necessity of integrating hardware and software development data, but at the same time, they recognize the difficulty that this process poses to traditional PLM architectures (Fig. 3).
“PLM systems typically do a poor job in managing software development lifecycles because they are just not able to cope with the dynamic, iterative processes used in software development, never mind methods like paired development or other software assurance tools,” says Thomas.
Furthermore, like IoT data management, software development involves large quantities of data. For example, in software development, the issues that come into play are the speed of development and the rate of change. For maintenance, it is the sheer volume of data that IoT devices generate. PLM systems simply were not designed from the ground up to handle these large data sets.
These data demands simply don’t match up with the capabilities of traditional PLM tools. As a result, PLM system developers are turning to new architectures to meet the latest challenges and limitations of their platforms.
A Federation of Equals
As part of their search for new architectures, PLM developers must first change their worldview. This means recognizing that PLM can no longer be a singular, one-dimensional application. Rather than being the center of the PLM universe, these platforms must become a member of a “federation of equals.”
Companies must reinvent how they organize their engineering departments, the processes they use and the systems that support these products. It calls for a much more systems engineering-oriented approach, driven by product functions and features rather than a product structure.
To gain access to the resources required to make these changes, users must transition from the one-platform mindset to one that is based on multiple best-in-class applications. The logic behind this move is that forcing a PLM system to incorporate various applications—such as an IoT or software system—means that the user must accept less-than-ideal functionality and all the handicaps that entails.
PLM, IoT and software development all have unique characteristics and requirements. To succeed, each system must be tuned to the unique needs of the people and use cases involved. That said, these best-in-class platforms must be tied together where use cases demand, enabling all of the systems to work together in a unified, holistic product development environment that can support complex use cases and achieve synergies.
Multi-Domain Integration Networks
One approach to the “federation of equals” paradigm offers a broad assortment of best-in-class domain and enterprise applications tied together via a common data backbone, leveraging standardized infrastructure capabilities. Systems based on this paradigm make applications available, on demand, over the web, via various deployment options. These range from on-premises to the cloud.
“One example of this can be seen in Teamcenter applications,” says Siemens’ Lewis. “Here, most of the deep connectivity mechanisms are shared and used across multiple types of deployments, using common foundational components. An example of this would be the configuration management engine. In this case, one configuration definition can be used to configure all the different data types, and because they are on a common data backbone, users can see exactly how that requirement affects the CAD design and how the CAD design lives in a bill of materials, and so on, throughout all the modules.”
Using this model, certain deployments may have a specialized implementation to address challenges like performance or cost. For example, a file management system may be optimized for cloud deployments, taking advantage of cloud-specific file storage systems provided by different cloud vendors.
To integrate third-party applications, this approach uses various integration mechanisms to plug the application into the larger PLM suite. These mechanisms range from simple linking of data between systems to replication and synchronization. The use case and needs of the application drive the direction taken for such integrations.
Looking to Native Cloud Systems
Another approach to the “federation of equals” paradigm takes a different tack, using an architecture based solely on native cloud systems—meaning the platform is built from the ground up for the cloud, with all associated applications connected to a cloud backbone.
A key distinguishing feature of these systems is their use of data services—self-contained units of software functions that organize, share or analyze information collected and saved in cloud storage. The inherent nature of these services makes information more accessible and comprehensible to a broad assortment of applications and adds characteristics to data that do not occur natively, such as metadata.
One benefit of using data services lies in their flexibility to handle configuration and customization of their data models.
“In the modeling and simulation domain, PLM software providers must deal with a plethora of tools—their own, third parties’ and client-built custom modeling tools,” says Thomas. “Unfortunately, most companies have limited to no governance to drive the consistency needed to feed this data into the PLM applications. To solve this problem, companies are increasingly turning to a distinct data services layer, which acts as a translator between the PLM platforms and the tools themselves.”
Data services’ information model further expands data accessibility by allowing multiple users to work concurrently on a design by breaking up the body of information into smaller pieces (e.g., one person works on the bill of material, while another works on the CAD files). This feature highlights the advantage of having all the data in the cloud in a single source of truth.
Combine data services flexibility and data management capabilities with the cloud’s storage capacity and computing resources, and you have a potential solution to many of the flaws pulling traditional PLM architectures toward obsolescence.
“By moving PLM systems to a cloud-based platform that leverages data services, management of associations between files and making sure everyone’s working from the current design becomes much easier to manage,” says Autodesk’s Welch. “Beyond the associative relationships, data service-enabled cloud PLM opens the door for a much more granular approach to data that’s simply not possible with on-premises solutions. Understanding how a metadata change affects an associated document or how a geometric change affects a process can all be automated with a modern, cloud-based PLM platform.”
More Autodesk Coverage
More Dassault Systemes Coverage
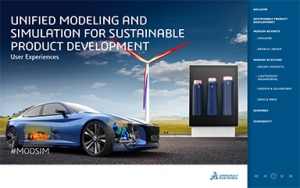
More Siemens Digital Industries Software Coverage
Subscribe to our FREE magazine,
FREE email newsletters or both!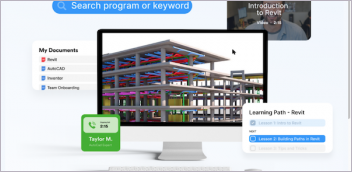
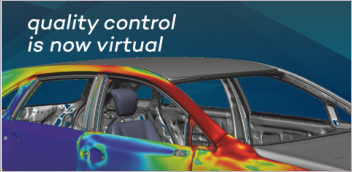
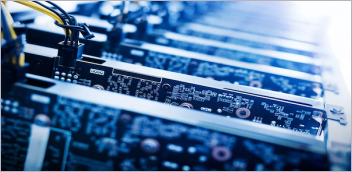
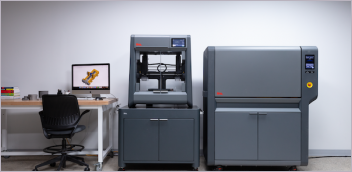