Latest News
February 1, 2015
By Barna Szabo
I recently visited an assembly plant of a major corporation. I was impressed with the efficiently coordinated activities, as well as the meticulous attention to quality and safety. I saw manufacturing governance in action.
My own field is computer-aided engineering (CAE) and I could not avoid noting the sharp contrast between what I saw in the assembly plant and the casual, even chaotic practices in simulation and analysis (S&A) that I have witnessed. Recognizing that S&A is increasingly important in guiding engineering decisions, I would like to make a case for simulation governance.
There are substantial economic incentives to reduce reliance on physical testing and increase reliance on numerical simulation. Testing is expensive and time consuming, and the results of physical experiments are tied to the specific conditions under which they are performed. Testing without planning on how the results will be interpreted and generalized makes no sense.
Using Mathematical Models
Reliable generalization of test results is possible through numerical simulation. Simulation is the imitative representation of the functioning of one system or process by means of the functioning of another. Let’s consider the functioning of mechanical and structural systems and their imitative representation by mathematical models. Mathematical models are also used for inferring information that cannot be observed in physical experiments.
The formulation of mathematical models imposes certain restriction on the input data. For example, point constraints are inadmissible in models based on the theory of elasticity, except when rigid body constraints have to be imposed.
Unfortunately, using inadmissible data can be intuitively plausible and can even produce credible numerical results. Nevertheless, the numerical solution will be an approximation to a non-existent solution and therefore useless.
How is this possible? Take the simple example of connecting two plates or shells by rivets or bolts. The analyst, not wanting to bother with the details of holes, bolts and rivets, simply assumes that it is OK to connect nodes instead. Rigid or spring elements are often used for this purpose. By using this intuitively plausible simplification, the analyst produces a numerical problem with a solution that does not correspond to a well-defined mathematical model. The numerical solution will be credible because the results will be close to what could be expected and the nodal forces will be in equilibrium with the applied loads. However, they will depend on the mesh.
Many analysts mistakenly believe that equilibrium of nodal forces is an indication of solution quality. The equilibrium of nodal forces is satisfied independently from the finite element solution. Even if we replaced the finite element solution with numbers from a random number generator, the nodal forces will be in equilibrium!
Finite Element Modeling vs. Numerical Simulation
The purpose of validation is to test the predictions based on a mathematical model against the outcome of physical experiments. When there is no mathematical model, validation is not possible. The analyst is practicing finite element modeling, not numerical simulation. This means that instead of thinking through how to simulate the physical reality of the object of interest by mathematical means, the analyst simply selects elements from an element library and connects nodes. He creates a numerical problem that does not correspond to a mathematical model.
How is it possible that even though there is no mathematical model, the results appear to be credible? There are two very large errors that nearly cancel one another. There is the conceptual error of using inadmissible data and there is a large error in the numerical solution. This makes it impossible to generalize the results of experiments outside of the range of experiments.
The purpose of physical experiments is either to calibrate or validate a mathematical model, not to tune a “finite element model” so that two large errors almost perfectly cancel one another. Therefore, one cannot generalize the results of experiments with justifiable confidence when there is no mathematical model to justify generalization. Finite element modeling is not numerical simulation.
Implementation is Key
Engineering organizations need to institute simulation governance. This means that management has to understand that finite element modeling is not numerical simulation and begin to exercise command and control over all aspects of numerical simulation. This includes adoption of the best available simulation practices, solution verification, data verification, model validation and revision of mathematical models in the light of new information collected from physical experiments. The primary goal of simulation governance is to ensure and enhance the reliability of predictions based on numerical simulation.
Barna Szabo is co-founder and president of ESRD, Inc. Contact him via [email protected].
More ESRD Coverage
Subscribe to our FREE magazine,
FREE email newsletters or both!Latest News
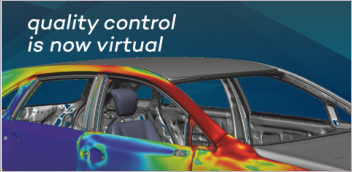
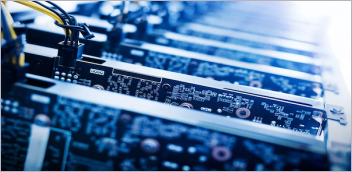
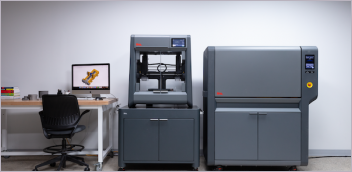
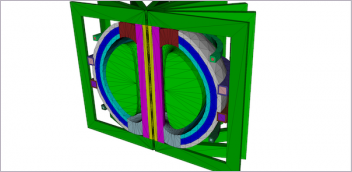