Using Synthetic Datasets to Train Embedded AI
This study demonstrates training and validating embedded AI algorithms using synthetic datasets derived from large numbers of parametric cloud simulations.

March 20, 2020
Using synthetic datasets to train AI is a fast, cost effective way to deploy robust embedded algorithms for new hardware technologies. Synthetic datasets generated from cloud simulations can be created in hours using the OnScale platform, compared to weeks or months to create similar datasets from physical experimentation.
This whitepaper discusses how an embedded AI algorithm for 3D smartphone touchscreens was trained and validated using the results of 8,000 simulations run in parallel on AWS. The demonstrated approach of using synthetic datasets to train AI networks can drastically reduce cost, risk, and time for the development of new hardware technologies.
OnScale is fully cloud-enabled, empowering engineers with the high-performance computing (HPC) resources needed to explore their design space quickly and with ease. Semiconductors, MEMS, sensors, medical devices, and 5G and IoT RF systems are among the many applications that can benefit from design and optimization with OnScale. To learn more visit onscale.com/applications.
Contents
- Problem: 3D touch technology operation
- Introduction: Using Synthetic Datasets to Train Embedded AI
- Approach: Simulating a synthetic data set & AI training
- Results: AI Performance
Fill out the information below to download the resource.
Latest News
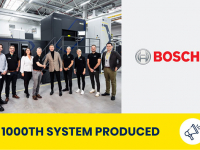
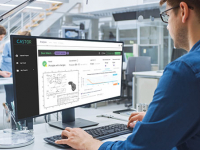
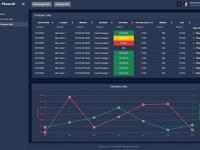
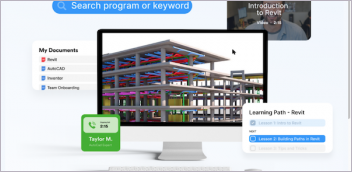
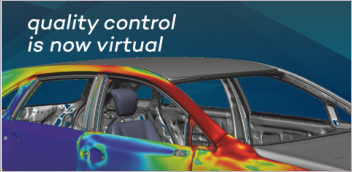
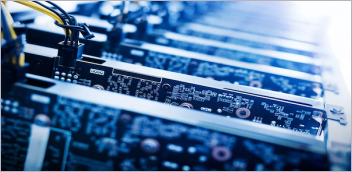
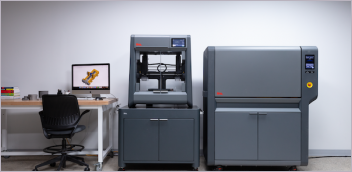