Visualizing Digital Twins
The true cost of deploying and maintaining a digital twin is in collecting and analyzing the data. The data gives life to static 3D models.
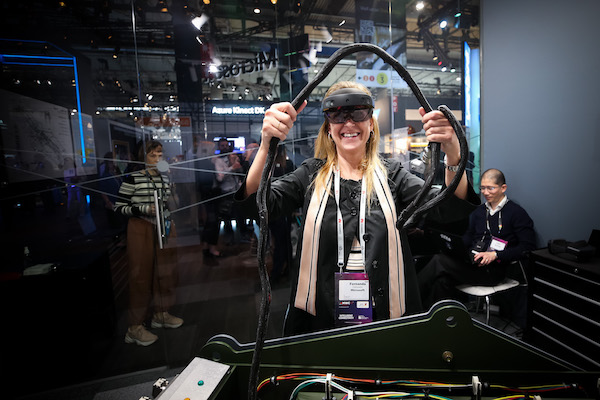
The NVIDIA Data Science Workstation, built to process and analyze large data sets, could be a critical component to the digital twin deployment strategy. Image courtesy of NVIDIA.
Latest News
December 5, 2019
In the last decade, the term “digital twin” began to pick up momentum, becoming part of the recognizable industry lexicon. The list of Microsoft Azure cloud offerings now includes Azure Digital Twins. Renowned analyst and consulting firm Deloitte published a paper on the role of the digital twin in Industry 4.0 transformation. Engineering software giants such as PTC, Dassault Systemes, and Siemens PLM began offering digital twin solutions, each assembling a collection from its existing portfolio to address the new phenomenon.
The term “digital twin” can easily be understood as a digital replica of a real product. For example, the digital version of a wind turbine or a power plant. But it’s a mistake to think of a 3D representation of the product as the digital twin. Such a model, usually derived from the detailed CAD model, is an incomplete digital twin.
A key component of the digital twin is data—historical data that allows users to study usage patterns and predict potential failures. Without it, the 3D model is nothing but a glossy snapshot of the product in its ideal state.
What is a Digital Twin?
In their paper titled “Industry 4.0 and the Digital Twin,” the authors Aaron Parrott and Lane Warshaw from Deloitte Consulting define the “digital twin” as “a near-real-time digital image of a physical object or process that helps optimize business performance.”
“Until recently, the digital twin—and the massive amounts of data it processes—often remained elusive to enterprises due to limitations in digital technology capabilities as well as prohibitive computing, storage, and bandwidth costs. Such obstacles, however, have diminished dramatically in recent years,” observed the Deloitte paper’s authors.
Some of the earliest references to the digital twin concept came from aerospace. In a paper titled “The Digital Twin Paradigm for Future NASA and U.S. Air Force Vehicles,” dated 2012, coauthors E. H. Glaessgen from NASA Langley Research Center and D.S. Stargel from the Air Force Office of Scientific Research defined the digital twin as “an integrated multiphysics, multiscale, probabilistic simulation of an as-built vehicle or system that uses the best available physical models, sensor updates, fleet history, etc., to mirror the life of its corresponding flying twin.”
In other words, the digital twin is aware of the real product’s installation date, maintenance history, downtimes, uptimes, and other major events in the timeline. Even its behavior in the digital world—how it responds to simulated wind, heat, pressure, and other loads—matches the real product’s behavior. With the digital counterpart as a stunt double (as it were), users can conduct what-if experiments on it without interrupting the real product’s critical operations, and without incurring significant risks of destruction.
The Unseen Costs of Digital Twins
The detailed 3D model of a product, down to the nuts and bolts, can easily be exported from the CAD model submitted in its final phase for production. This process involves little or no extra cost. But the true cost of deploying and maintaining a digital twin is in collecting and analyzing the data.
To deploy the digital twin of a wind turbine, for example, you would need to equip the product in the field with sensors to collect daily wind speed, weather data, moisture data, power usage, and other information. Depending on the volume of the data, a couple of data scientists or a team may be required to monitor and study them. Without this process, the historical usage data is merely a record of events without any predictive value. Only by devoting competent data scientists can you expect to be able to identify common patterns, such as those that indicate impending failures.
The cost and operations involved in launching and maintaining a digital twin is not trivial. Therefore, it makes more sense to deploy digital twins for high-end products with a long lifespan, indispensable to daily operations and difficult to replace. Creating digital twins for every unit of espresso machine sold, for example, may not be the best use of the technology. On the other hand, a digital twin for a passenger plane or an autonomous vehicle can easily offset its cost with the safety benefits it offers and the increased operational lifespan it enables.
The Data Scientist’s Workstation
Introduced by GPU maker NVIDIA at its GPU Technology Conference (GTC) in 2019, the Data Science Workstation targets those who must wrestle with Big Data—the type of data-driven analysis and simulation common in developing IoT products. The workstation combines the processing power of Quadro RTX GPUs with accelerated CUDA-X AI software, offering sufficient computing resources in a compact desktop form factor. This could be the centerpiece for teams monitoring and managing digital twins. Various versions of the Data Science Workstation are offered by NVIDIA’s workstation partners, such as Dell, under their own brands.
AR-VR for Digital Twin Visualization
Previously, visualizing digital twins means looking at CAD models along with tables of data, usually in separate software programs. The affordable AR/VR (augmented reality, virtual reality) systems now offer the option to visualize digital twins in a much more comprehensive fashion. With the ability to interact with the model in virtual environment, the digital twin can now be used for realistic training and simulation.
According to a case study published by PTC, industrial equipment manufacturer Howden has been using AR applications created with PTC’s Vuforia Studio software and IoT platform ThingWorx to let its customers virtually “see” what’s happening inside the products.
“Howden is pulling ThingWorx data operating on an Azure IoT Cloud and overlaying it on the physical product. This is to show their customers, in context, the operating conditions, and performance of the equipment to improve day-to-day operation. Predictive maintenance alerts, rapid parts identification and easy to follow repair sequences provide all the information relevant to resolving problems and keeping the equipment running as efficiently as possible to prevent failures and downtime,” writes PTC. For visualization and interaction with the model, Howden uses Microsoft HoloLens. Many AR-VR devices demand a powerful workstation with professional-grade GPUs.
Currently there’s no standard device or software for viewing digital twins. The task involves a mix of large datasets (usually in comma-separated databases), 3D models, and simulation programs. It’s a void that AR/VR system developers can potentially fill.
More Dell Coverage
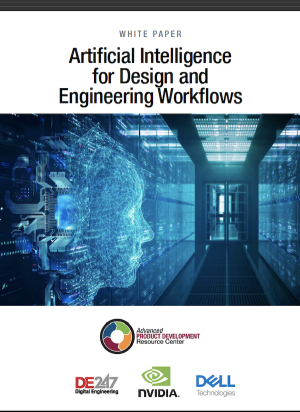
More NVIDIA Coverage
Subscribe to our FREE magazine,
FREE email newsletters or both!Latest News
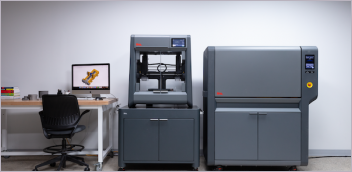
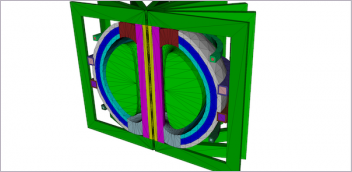
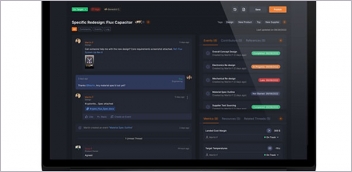
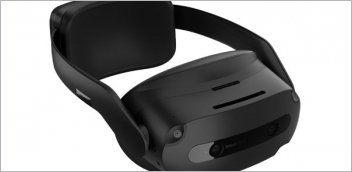