Simulating the Way to Consistent 3D Printed Parts
As production-scale AM gains traction, process simulation and in-situ monitoring are keys to consistent quality and optimized performance for 3D-printed parts.

Process simulation capabilities in 3DXpert help prevent costly build failures. Image courtesy of Oqton.
Latest News
May 16, 2023
Additive manufacturing (AM) has made significant inroads as a viable alternative for production-grade part creation. Yet even with advances in 3D printing hardware as well as software aimed at optimizing designs for AM output, demand is growing for tools capable of monitoring and simulating AM processes to ensure consistent and repeatable quality and part performance.
The gap is starting to close as companies roll out new process simulation and in-situ monitoring offerings that give 3D printing professionals valuable insights into how to modify print parameters and material properties to achieve high-quality parts at scale. It’s just not practical to take the time and spend the resources to iterate the perfect set of parameters for printing a handful of bespoke parts or prototypes. That process simply doesn’t hold up from an efficiency or return on investment (ROI) standpoint when orchestrating limited or full-scale manufacturing runs.
Many companies getting started with AM expect the process to be linear, from designing a part to printing it locally, all within a relatively short time span.
“The reality is quite different—there are areas where iterations are just prevalent,” says Doug Kenik, director of product management for software at Markforged.
Kenik was previously CEO at Teton Simulation, which was acquired by Markforged last April. Its cloud-based simulation software, which automates validation and optimizes AM part performance, is now integrated into the Markforged Digital Forge metal and carbon fiber AM platform.
Historically, it’s been somewhat of a guessing game to evaluate a 3D printed part’s ability to perform as specified, but simulation, including process simulation software, changes that equation.
“What a lot of people do now is throw a dart and see if something works and if not, they go back and redesign it or change something about the print and try again,” Kenik says. “The other option is to print it solid, but that is a waste of material and time. Simulation solves that problem—it will tell you if something will work or not before you print it, instead of physically iterating through printing and testing.”
While topology optimization and generative design software elevate the ability to tailor designs for AM and new process monitoring tools help engineers perform layer-by-layer inspection of the build process, there is still a wide delta between collecting all that data and leveraging it to actually produce reliable, high-quality parts consistently. The problem largely lies with lack of integration between the different software tools and 3D printer platforms, says Brad Rothenberg, CEO of nTopology, which markets engineering design software fine-tuned for AM.
A direct implicit connection between nTopology and EOSPRINT enabled Siemens Energy to manufacture a previously unprintable industrial heat exchanger. Image courtesy of nTopology.
“The pieces are all there—the core issue is how those pieces connect together,” he says. “Specifically with process monitoring tools—we’re collecting all the data, but the issue comes down to how to use the data and make it actionable. How do we learn from that data to make better and faster design decisions and create products that are more reliable, with better performance, at less cost?”
New Offerings on Deck
Materialise is addressing some of these issues, most recently with a new process monitoring solution for metal 3D printing along with a toolkit used to modify a printer’s build parameters to achieve more consistent quality. Both offerings are designed to help AM users avoid the failed builds, hidden defects and standard 3D printing parameters that aren’t a match for their AM applications and which result in wasted machine time, material and post-processing capacity.
Materialise Process Control, integrated with the CO-AM 3D printing software platform, uses AI to automate quality control. Image courtesy of Materialise.
“Ideally, a printer will produce the same component time after time, but with some components, that’s not the case,” says Bart Van der Schueren, chief technology officer at Materialise. “All kinds of things can happen while depositing the powder layer and it’s oftentimes something no one can see during the process.”
Materialise’s new Process Control solution uses artificial intelligence (AI) to analyze and correlate layer data from the 3D printing process, helping users identify defective parts before they are sent to post-processing or quality inspection, which the company estimates can account for 30% to 70% of a part’s total manufacturing cost. Through collaborations with Phase3D and Sigma Additive Solutions, both providers of in-situ AM process inspection solutions, Materialise is integrating complementary data that will develop deeper insights into the 3D printing process.
The Phase3D partnership adds height mapping, a form of topographic layer data and Sigma Additive Solutions delivers thermal data from the melt pool to optimize 3D print quality.
Materialise also announced the Build Processor software development kit (SDK), which delivers an open development platform for machine builders and customers so they can more easily create and tailor build processors to hit their specific sweet spot between cost efficiency and part quality in support of mass AM production.
Van der Schueren says the most valuable information users can glean from process monitoring is not necessarily an in-situ correction on a layer-by-layer basis, but rather to examine the totality of images and take a global view. It’s also important to evaluate the data and insights as part of a holistic platform as opposed to individual silos, he says. To that end, the Process Control solution and future offerings have full connectivity to the company’s CO-AM open software platform for managing the full AM process.
“You have to have a holistic view on the total process and take everything into account starting from data preparation up to the file component and part inspection,” he says.
Hexagon Manufacturing Intelligence is leveraging simulation and modeling to approach the AM parts quality problem. The company has collaborated with printer manufacturers to validate the combination of specific materials and AM processes as part of its Digimat multiscale material modeling platform.
In one such example, the firm partnered with Stratasys to capture the behavior of Stratasys’ high-performance, lightweight Antero reinforced polyetherketoneketone (PEKK) materials and its specific AM processes. This effort gives AM customers validated simulation models that help predict how parts printed with PEKK and approved Stratasys printers will perform without having to physically incur the time and costs of physical prototyping.
On the metals side, Hexagon Manufacturing Intelligence has partnered with Raytheon Technologies on a multi-scale simulation tool that will complement its Simufact Additive process simulation software and be made available in November.
Combining technologies from Hexagon and Raytheon’s Pratt & Whitney business, the partners are developing a tool that predicts thermal history and defects at the laser path and powder layer scale to test combinations of materials, geometries and print processes to achieve better print outcomes and part integrity, according to Mathieu Perennou, Hexagon Manufacturing Intelligence’s global strategy and business development director, additive manufacturing.
“This enables users to change processes for the better as well as give feedback to the models to calculate more accurate part performance,” Perennou says.
The Hexagon portfolio also features Volume Graphics’ VGSTUDIO Max, which performs computed tomography scan analysis on printed parts for quality inspection, ensuring defects like porosities are identified promptly. This part of the workflow also allows for the porosity map to be “injected” into an finite element analysis (FEA) model for further part performance analysis, Perennou says.
Looking Toward the Future
Though AM process simulation at macro-scale levels allows for manufacturability checks, it doesn’t currently provide ample data on the quality of material produced—a critical and unique element of the AM process and a hurdle limiting AM adoption, especially in highly regulated industries like aerospace.
“A micro-scale simulation will give accurate information on the quality of the material made during the printing process, but such FEA approaches are very CPU-intensive, requiring long solving times,” Perennou says.
As of now, “they are typically performed at a coupon level and are therefore challenging to scale to a complete part in an industrial environment.”
In fact, the massive data sets collected by process simulation tools are one of the core bottlenecks to making insights actionable as a way to fine-tune AM processes, says Daeho Hong, product manager at nTopology.
He envisions a future where in-situ monitoring capabilities are used not just to suggest or automate changes to 3D printing processes or tool paths, but rather applied at the design level. To support that type of workflow, complex part geometry data has to be integrated directly onto the 3D printer hardware without the need for meshing—a use case primed for nTopology’s implicit modeling capabilities, he says.
“Imagine a full design and manufacturing digital thread so tightly coupled that what you learn from the design of experiments could be fed back into the design,” Rothenberg explains. “Dealing with large data sets is the critical problem that needs to be addressed.”
nTopology is currently working with Siemens Energy on an interoperable data exchange mechanism that leverages its implicit design capabilities to transfer complex geometry in a light and scalable way to EOS printers. The capability, which will be made available to nTopology customers, is currently being expanded to support additional 3D printing hardware in the future, he says.
Eventually, it will be important to close the loop so that the in-situ process monitoring insights can automatically and directly impact 3D printer parameters and performance. However, proprietary printer architectures and the relative immaturity of current technology makes that difficult at this point.
“It’s a chicken and egg problem—every machine manufacturer has a different approach and the sum of those approaches creates complexity,” says Fabian Grupp, product marketing manager for industrial AM at Oqton.
In the interim, taking a holistic approach enabled through a hybrid design for AM environments like 3DXpert combined with the Oqton Manufacturing Operating system can help organizations maximize consistent AM output through capabilities that include designing support structures, simulating builds, calculating scan paths and optimizing arranging the build platform. Beyond any technology investments, organizations must commit dedicated resources to AM and enlist management buy-in at the highest levels.
“You need dedicated resources for rolling out AM as a full-blown manufacturing strategy with successful scalability,” Grupp says.
More Hexagon Manufacturing Intelligence Coverage
More Markforged Coverage
More Materialise Coverage
More Oqton Coverage
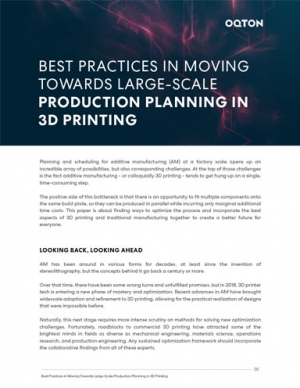
Subscribe to our FREE magazine,
FREE email newsletters or both!Latest News
About the Author
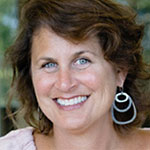
Beth Stackpole is a contributing editor to Digital Engineering. Send e-mail about this article to [email protected].
Follow DE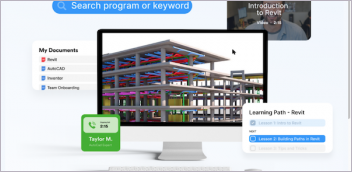
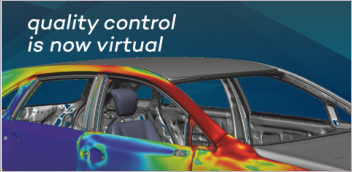
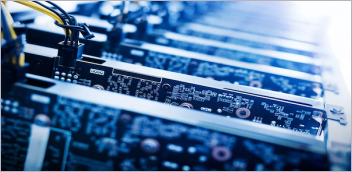
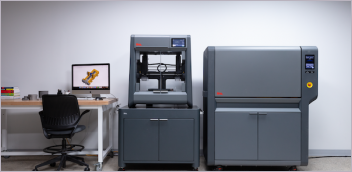