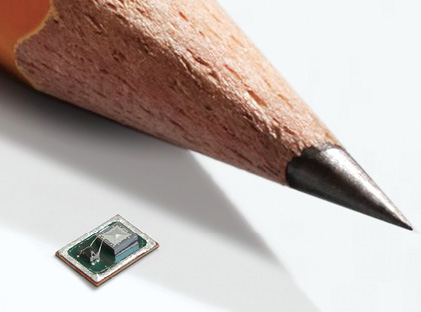
Smart microphones have made it possible to incorporate voice interfaces in mobile and wearable devices, dramatically changing the way users interact with computing systems. Image courtesy of Vesper Technologies.
Latest News
February 1, 2018
A device the size of a pencil point has brought the voice interface to the verge of mainstream consumer electronics, with the control mechanism poised to transform the way people interact with machines. Smart microphones have opened the door for interfaces that adapt to human behavior better than any previous technology—surpassing both touch- and gesture-based interfaces.
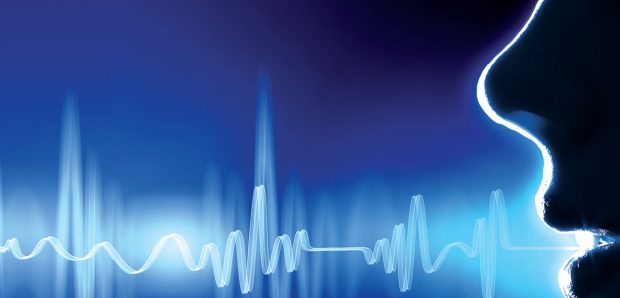
Advances in acoustic sensing and signal processing techniques now enable these tiny devices to support human–machine interactions across rooms. Smart microphones represent the fundamental technology upon which voice interfaces are built. To understand and interpret speech reliably, the interfaces must hear just as clearly as people do. This means that the devices must be able to filter out extraneous noise and emulate the directional hearing that people do naturally.
A scan of today’s market reveals the microphone’s strong presence in products ranging from smartphones and wearables to smart appliances and home hubs. To see the extent of their market penetration, consider the statistics: Amazon Echo already resides in 4% of American households; Apple’s Siri responds to more than 2 billion commands a week; and 20% of Google searches on Android smartphones in the U.S. are done via voice commands.
Part of a Larger System
When people talk about “smart microphones,” they are actually referring to an array of microphones, supported by special signal processing hardware that helps to locate and isolate speech. With each new generation of voice-recognition technology, the number of microphones in the arrays has increased. For example, the first iPhone had one microphone, but the iPhone 5 had four sensors. Devices like Amazon Echo use as many as seven microphones.
These microphone arrays can be made up of omni-directional microphones, directional microphones or a mix of the two, distributed in a variety of configurations. As a sound wave passes over an array, the wavefront reaches each microphone at a slightly different time. The system uses the time difference to triangulate the origin of the sound.
But there is much more to the process than triangulation. Voice-recognition systems begin with the microphone array converting acoustic waves to electronic and then digital signals. The array passes the digital signals to a digital signal processor, where speech enhancement is applied via specialized algorithms. This processing involves locating the direction of sound and contending with a number of factors that can compromise the acoustic signal. Finally, the enhanced signal goes to a conventional acoustic model for speech recognition.
To achieve optimal performance from a microphone array, the designer must closely match the sensitivity and frequency response of each microphone. Differences in these two parameters among the various microphones can cause a breakdown of the array’s desired response.
Two Sensing Approaches
Current microphone technology includes old and new approaches. Capacitive micro-electro-mechanical system (MEMS) microphones have traditionally populated microphone arrays. This type of microphone uses a diaphragm and one or two back plates to form an air-gap capacitor that has a high bias charge. When the diaphragm moves in response to sound waves, the capacitance changes and the resulting voltage increases.
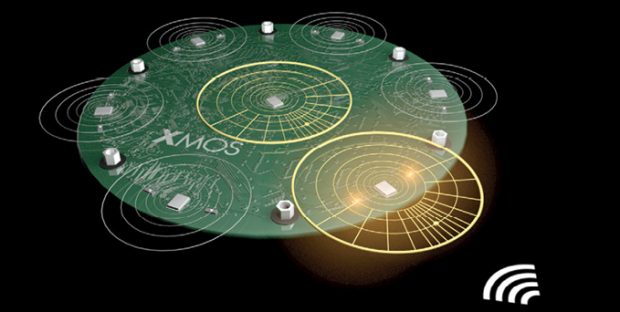
High-performance capacitive analog MEMS microphones deliver 63–65 dB SNR, as well as clear audio pickup, and the device offers a small footprint. On the negative side, however, these devices often succumb to the wear and tear of harsh environments. Capacitive microphones tend to be easily damaged by contaminants like water and dust. If even one microphone’s performance is compromised by environmental conditions, the performance of the entire array deteriorates.
Despite this flaw, capacitive microphones have long dominated the market, and most major microphone vendors rely on the technology. On top of that, leading device manufacturers like Apple use capacitive MEMS microphones in their mobile products.
Recently, however, vendors have begun to introduce piezoelectric MEMS microphones, which offer impressive advantages. The developers of these devices have replaced the diaphragm and back plate found in capacitive sensors with a design that allows the sensing device to withstand harsh conditions without workarounds to ensure high reliability.
Piezoelectric MEMS microphones are relatively new to the market, but the strengths that they bring to the table have captured the attention of major developers like Amazon. Last year, Amazon’s venture capital arm, the Alexa Fund, announced that it had contributed to Series A funding for Vesper, an early provider of piezoelectric MEMS microphones.
In Search of Clarity
Whether the microphones rely on capacitive or piezoelectric technology, the arrays enabling voice interfaces still have their work cut out for them. Typically, voice interfaces operate in noisy environments, cluttered with competing speakers, background noise and audio system reverberation that masks or corrupts the desired speech signal. Before the interface can process natural language, the microphones must sift through a flurry of acoustic signals, identify the source of interest, determine the direction from which it is projected and mitigate the effects of undesired signals and noise.
One of the most useful technologies in the developer’s toolbox for addressing these issues is “beamforming,” a process that extracts sound from a specific area of space. The beam usually takes the form of a plane or cone-shaped segment of space. Engineers measure beamforming’s effectiveness by how much of the signal outside the beam is eliminated and how well the microphones focus on a speaker’s voice by steering the directivity pattern toward the source. So not only does beamforming allow the microphone array to focus on the desired voice, but it also eliminates unwanted noise.
Voice interface designs must also contend with acoustic echo and reverberation. Acoustic echo stems from audio-playback paths often found in voice-recognition systems. The echo occurs when microphone elements of the array pick up the playback audio and feed it back into the system. This problem becomes particularly bad when the audio playback volume is high or when the speakers and microphones are close together.
Reverberation, on the other hand, occurs when audio-signal reflections from furniture and structural elements—such as walls—are fed into the microphone.
To mitigate the effects of these conditions, designers use acoustic echo-cancellation algorithms and de-reverberation filters. Recent efforts to eliminate interfering noise, however, have moved beyond these traditional approaches. Increasingly, voice interface designers rely on machine learning to clean up the noise clutter and drive further enhancements. They achieve this by using deep neural networks to block all but the desired signal.
From Design Parameters to System-Wide Concerns
The relevance of beamforming, acoustic echo-cancellation algorithms, de-reverberation filters and deep neural networks depends on the operating environment in which the microphone array must function. As you might expect, for these applications, that translates into parameters of space and operating distance.
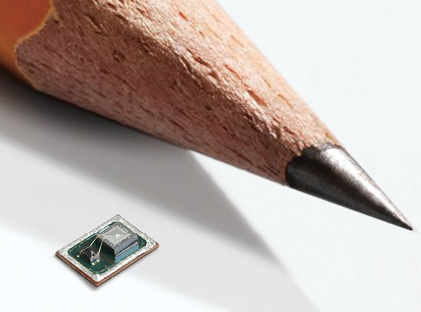
There are two broad types of voice-based interfaces: mobile and wearable device interfaces, which typically have to function over short distances, and smart home hubs like Amazon’s Echo or Google’s Home, which must function over longer distances.
“To date, yes, these two types dominate, and maybe there will be even longer distances in the future,” says Matt Crowley, CEO of Vesper Technologies. “The design requirements of mobile and wearable lend themselves more toward ruggedness and high acoustic overload for things like wind noise because it’s associated with outdoor environments. The design of a hub-type device also demands good far-field performance—since the audio source may be far away—and also high acoustic overload for things like barge-in if the hub is playing music at the same time. There is a large amount of overlap in desirable acoustic specs, but the driving reasons are slightly different.”
That said, some parse voice interface applications into more subcategories. “There are three different types of voice interface: hand-held, hands-free and far-field,” says Huw Geddes, director of marketing for XMOS. “Hand-held includes wearables, mobiles, etc., where users [are] in the 10–50 cm range. Designers can make informed judgments on the position of the voice source and predict the relationship of microphones and voice source. Hands-free includes devices like an intercom or speakerphone, where the user is in the 100 cm range. Like hands-free, designers make informed judgments on the position of the speaker. Then there are far-field interfaces, which need to be able to capture voice across the room in the 2 to 5+ meter range, and in environments where the voice source can be in free space and moving. In this case, designers cannot make judgments about the relationship of speaker and microphones.”
Short-Distance Interfaces
As you can see, the operating range of short-distance interfaces sets some of the design parameters for products that include smart microphones. But development teams must also consider other short distance-specific and system-wide issues.
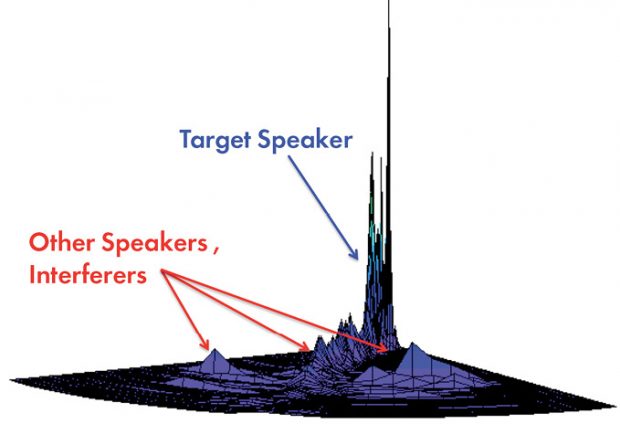
For example, noise cancellation plays a large role in the effectiveness of the interface and the quality of the user’s experience. Smart microphones should be able to cancel all known noise sources encountered by mobile, wearable and hands-free products. To this end, the designer should bear in mind that microphone placement—which affects frequency response and directivity of any beamformer—greatly determines a microphone array’s ability to effectively eliminate noise. This task poses unique challenges in space-constrained designs exposed to demanding physical environments.
But perhaps the most unique challenge encountered in the design of this group of products is power management. For smart microphones, this issue is often linked with always-on functionality. “Power consumption in mobile and wearable devices is critical,” says Todd Mozer, CEO of Sensory Inc. “Low-power, sound-detection circuitry creates an ultra-low power consumption for always-on, always-listening devices by awakening the recognizer only when interesting audio is present and then following with a very low power recognizer.”
Another factor that plays a key role in ensuring a seamless user experience is latency—how quickly the interface responds to the user. “Existing digital signal processing subsystems on the SoC (system on a chip) for noise suppression and cloud communication already add to the overall latency,” says Crowley. “Therefore, a smart microphone, if needed, should be chosen to optimize the latency of the system.”
In addition to these two issues, speech processing must also be tailored to the applications. This raises a number of questions that the designer must address.
For instance:
- What type of signal processing is required? Are multiple input channels needed for speaker localization, beamforming or noise profiling?
- Is the audio quality sufficient for speech processing? For example, nonlinear processing may negatively interfere with voice user interfaces.
- Are any speech-processing related functions available on the smart microphone hardware, such as speech-activity detection? Are any of these, or lower-level functions like an FFT (fast Fourier transform), implemented in silicon?
These are just some of the issues development teams must factor into their designs. And because it is still the early days for voice interfaces, the systems will only become more complex.
Far-Field Smart Microphones
Designers of far-field microphone arrays face a different set of problems than those encountered by developers of short-distance arrays. Many of the differences emanate from the acoustic properties with which they must contend. At the same time, developers have the option of using different techniques from their short-distance counterparts. As a result, design engineers beginning a far-field project must take a different perspective.
“It’s important to remember that sound behaves differently over different distances,” says Geddes. “Sound waves near a hand-held or hands-free device are spherical, which makes it easier to identify the location of a sound source. With far-field microphones, the sound wave is usually assumed to be planar, which means that different simpler algorithms can be used to identify the source location. In addition, voice sources distant from the microphone are quieter and often have to compete with other surrounding noise sources, including other electronics and people.”
In addition to these issues, design engineers need to be particularly aware of the placement and decoupling of speakers relative to the microphones. The output volume is limited by the acoustic echo cancellation’s ability to remove the speaker signal. Preprocessing can help, as well as better speakers with less total harmonic distortion.
Designers should also pay attention to the impact of the system’s enclosure on acoustic performance. The positioning of the microphones has a significant effect on the ability of the device to cancel out bouncing signals and reverberation, identify direction of arrival of the voice signal and capture the loudest possible signal.
The number of microphones in the array and the use of automatic gain control also affect performance. “More microphones are needed for better sound processing,” says Mozer. “Also, the microphone should allow for real automatic gain control, either with hardware amplification or with 24–32 bits of information that allow shifting up or down for the 16-bit window that represents the target audio.”
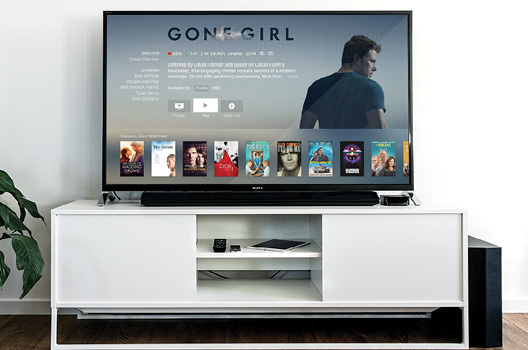
Beamforming also plays an important role in this class of application. Smart microphones based on beamformers enable arrays to identify real human voice sources (as opposed to voices on the TV) within a noisy soundscape at a distance of several meters. There are, however, types of smart microphones based on different mathematical algorithms that can deliver similar results.
Given the scale of the geography within which far-field arrays must perform, smart microphones in these applications must be able to function well in all kinds of conditions. “The ability of the system to adapt to different environments and climatic conditions is important,” says Geddes. “Devices often work differently in winter vs. summer conditions, or even in the same location at different times of the day. A device optimized to handle reverberation well might work efficiently in an office environment, but much less effectively in a room with many soft furnishings as the voice signal is not strong enough. So the solution must be flexible and able to be re-tuned, maybe using some type of AI.”
Looking at the Big Picture
It’s important to remember that smart microphones do not operate as discrete systems. Optimal design or selection of smart microphones cannot solely rest on the issues outlined. A more holistic view of the increasingly complex and interconnected components and subsystems making up voice interfaces must also be considered.
This broader view requires designers to consider other factors. Speech and language applications can vary in many aspects, some of which are listed below.
- Does the application run on the device or does the cloud come into play? Is it a hybrid, leveraging onboard and server-based speech and language technology?
- Is the interface an embedded unit or a larger one? Is it battery operated or does it have a constant power supply? Can parts of the speech processing be done on dedicated hardware, such as a DSP or neural network hardware acceleration unit?
- What part of the world does a speech recognition service cover? Is it limited to a dedicated domain, such as transcribing radiology reports, or is it a voice assistant with large coverage? Is it available in a single language or in multiple languages?
- Is the interface a command-and-control system, with a small set of distinct commands (e.g., a set of commands for warehousing that can be understood robustly even in adverse acoustic conditions), or is it a natural language application like a virtual assistant that needs to deal with flexible, natural language?
Balancing these elements to meet design requirements means making the inevitable tradeoffs. “If the device will be used in noisy environments, microphone arrays can deliver multichannel audio that can be used for beamforming to home in on the speaker’s voice,” says Holger Quast, senior principal product manager, Nuance Communications. “If this is used in an always-on recognition mode like with a wake-up word application, power consumption may be an issue if it’s a small battery-operated device, so possibly DSP hardware solutions may help. If other modalities like a visual channel (camera) are available, that information may also be used to locate the user and set the beamformer.”
More Info
Subscribe to our FREE magazine,
FREE email newsletters or both!Latest News
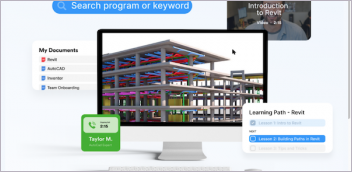
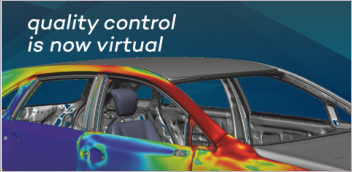
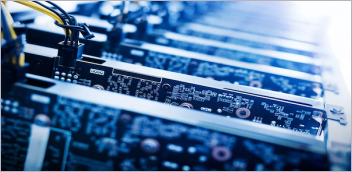
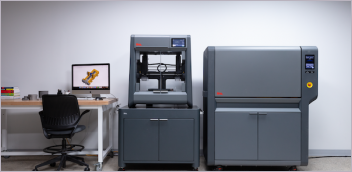