Roadblocks Slow the Digital Twin Race
Latest News
May 1, 2018
Although an engine tweak or a small part change can deliver the win, the frenetic pace of the NASCAR race season means a good idea has a shelf life of only about a week or two. To accelerate its process, Hendrick Motorsports is throttling up a digital twin effort to test drive changes quickly before building physical prototypes and to leverage real-time engine performance data to fine-tune winning designs.
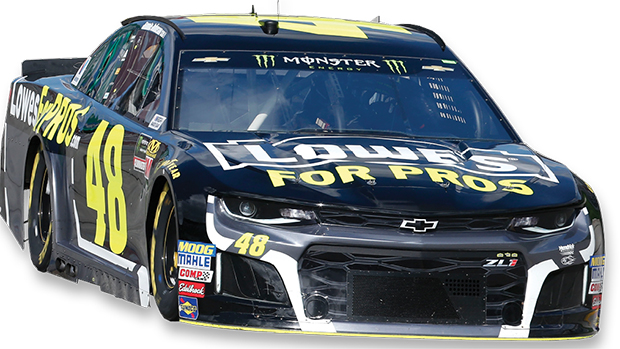
“Our schedule is pretty rigorous with 36 race events a year, which means we are reinventing ourselves every week,” explains Jim Wall, Hendrick Motorsports’ senior director of engine programs. “The digital twin gives us the freedom to explore design options and variations without committing to a physical model.”
Like at Hendrick Motorsports, the digital twin is starting to take shape at manufacturers that are building everything from cars to aircraft to wind turbines. Hendrick Motorsports got an early jump and lapped the concept for its powertrain components, but it hasn’t yet crossed the finish line on a complete digital master, Wall says. Other front-runners are also pacing digital twin efforts, hitting the brakes for a variety of reasons, including varying interpretations of the concept, differences in scope and the lack of a packaged toolset to create a holistic digital twin model.
“There are lots of variations in interpretation and definitions of what a digital twin is, and it’s lumpy in terms of the maturity of models,” notes Jonathan Scott, chief architect at Razorleaf, a PLM consultancy. “Organizations may not have the whole product ready, but certain parts of it are really advanced in terms of their digital ‘twinness’ because of the business needs.”
Mixed Interpretations
There is universal enthusiasm for the concept of a digital twin as a bridge between the digital and physical worlds as well as plenty of talk about its potential for creating feedback loops to advance product designs, optimize the operation of industrial assets and enable new use cases in areas like predictive maintenance. Spurred by the rise of internet of things (IoT), Gartner predicts that half of large industrial companies will leverage digital twins by 2021, resulting in those organizations gaining a 10% improvement in effectiveness.
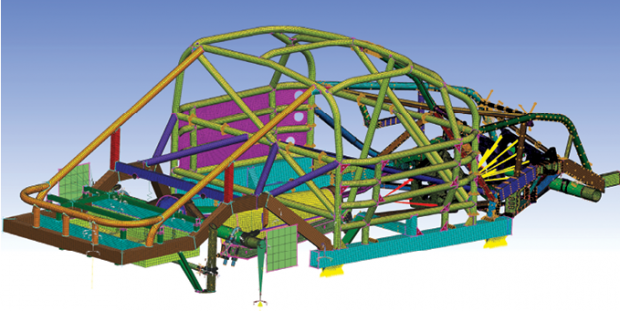
Yet even Gartner indicates that the complexity of digital twins will vary based on a variety of factors, including use case and business objectives, along with the specific vertical industry. In some cases, a digital twin will constitute something familiar like a high-fidelity, physics-based digital model based on 3D CAD and CAE analysis. In other scenarios, the digital twin interpretation is even more complex, becoming a composite of multiple digital twins integrated at a systems level and including process behavior that spans activities throughout the product lifecycle, including manufacturing, the supply chain and in-field performance data.
At Siemens PLM Software, the digital twin is viewed in the context of a lifecycle view—a virtual representation of a physical product, its production processes and its performance. In addition to traditional 3D models from CAD and CAE systems, Siemens is facilitating the creation of a digital twin through a variety of other solutions in its portfolio: Amesim, a multi-domain platform for system-level modeling and analysis, including software-in-the-loop and hardware-in-the-loop testing; Xpedition, for modeling PCB design flows and electrical hardware; Polarion, for managing complex configurations of embedded software through application lifecycle management (ALM); and Teamcenter as a central repository and workflow engine for managing the requirements, functional, logical and physical (RFLP) aspects of a product.
“The greatest value of a digital twin is when you can use the information and insights gained from a product, plant, asset or operations to improve its performance or the design or to leverage simulation to predict future performance,” notes Tom Maurer, Siemens’ senior director in strategic communications.
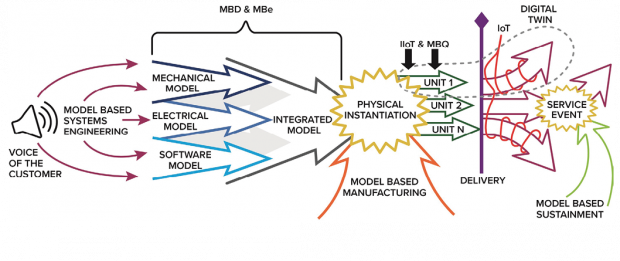
Dassault Systèmes uses a broad interpretation of the digital twin concept. What it calls a 3DEXPERIENCE digital twin is a virtual representation of the product used to understand how it will serve its need as it was designed—but also to explore how it will be manufactured and delivered to market so the entire value chain can be optimized to operate most effectively, according to Eric Green, vice president of user experience marketing for Dassault Systèmes’ DELMIA brand. Dassault users would leverage CATIA, the SIMULIA simulation solutions and DELMIA digital manufacturing tools to create a 3DEXPERIENCE digital twin.
In Dassault Systèmes’ vision, for example, a 3DEXPERIENCE digital twin could be called into play when a new automobile design is slated for production in a particular plant. “Say you have a large automobile being manufactured in a plant that is now slated to produce a small automobile model,” Green explains. “That has a direct impact on space and the movement of the assembly line. By modeling the product, the factory and the manufacturing processes, you can determine how everything behaves during production, using simulation and optimization to make the procedure most effective without expensive trial-and-error dry runs.”
PTC’s interpretation of a digital twin also includes manufacturing and supply chain as part of the digital product definition, but its concept is predicated on IoT and the notion of a connected product that captures performance, environmental and other data specific to its operation in the field. “Without the physical experience of the product, you don’t really have a digital twin,” says Mark Taber, PTC’s vice president of marketing, explaining that it’s the usage and error code data, for example, that after proper analysis, can really provide value to different stakeholders in manufacturing, engineering and service when used in context with the rest of their data.
PTC also believes that the entire digital twin isn’t necessarily relevant to everyone—that a solution should serve up different variations relative to an individual’s role and use case. Key to this strategy is the company’s Navigate technology, which taps into different systems of record like PLM, ECAD systems or simulation platforms and serves up the relevant information in a role-based app. “There’s one digital twin but different views of the digital twin depending on the role and what kind of information is necessary for the job,” explains François Lamy, vice president, solutions management, for PTC’s IoT Solutions Group.
“You don’t need a complete definition of a digital twin to get some value out of it,” he adds. PTC is currently working with customers to pilot Navigate digital twin apps, but has not made any formal announcements.
Overcoming Obstacles
It’s certainly possible that companies will engage different digital twins with varying degrees of fidelity for different types of applications—for example, leveraging IoT data for predictive maintenance or a high-level model for refinement of supervisory control and data acquisition (SCADA) systems, explains Jim Tung, a fellow at MathWorks. Companies can create a digital twin to monitor the behavior of a specific, serialized asset like a wind turbine or can also expand the use case to multiple digital twins of several like individual assets (such as a wind farm), treating them as a population upon which they can direct operations in a fleet management capacity.
“Customers are implementing digital twin strategies starting with what their objectives are for a particular asset,” Tung explains. “Those business objectives are driving the kind of digital twin to implement … because it’s an awful lot of baggage to carry around models of everything.”
In addition to highly advanced algorithms and machine learning capabilities, companies are making strides to better understand the data necessary to drive their specific digital twin application while solutions like MathWorks’ MATLAB and SIMULINK, along with CAD and simulation packages, have been enhanced to work more directly with big data.
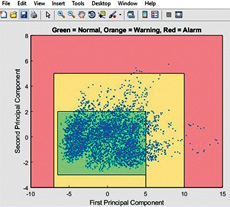
“What we see are companies being more refined in understanding what data they need to keep, where they need to keep it and where in industrial IoT they want to process it,” Tung says, adding that automatic code generation and the ability to integrate to enterprise systems like Hadoop databases provide additional flexibility.
Data challenges aside, aggregating and integrating the different models to create a holistic digital twin is likely one of the biggest challenges to implementation. Because there is so much variety in the type, complexity and number of systems required to manage and model, much of the effort to create digital twins is currently done in a do-it-yourself (DIY) fashion without the benefit of purpose-built, off-the-shelf frameworks or software functionality, experts say.
It’s a gap ANSYS hopes to address in the next iteration of its simulation platform, according to Eric Bantegnie, the company’s vice president and general manager of the systems business unit.
Although ANSYS currently has a wide number of simulation tools for addressing the different physics areas of the digital twin (mechanical, fluid and engine controls, among many others), it, along with leading 3D tools providers, is currently lacking functionality to make it easier to integrate disparate models into a holistic digital twin.
“The digital twin is nothing more than the optimal integration of a variety of simulation models into a systems-level framework,” he explains. “We need to extend our multiphysics product line with a solution that aggregates all simulation models to create a system-level digital twin.” To that end, the ANSYS Twin Builder, part of its next major release, will allow design teams to organize all of the disparate simulation models comprising a digital twin—FEA, CFD, electrical, embedded software, for examples—into a systems-level digital model that depicts the characteristics and behavior of a physical offering.
Back at Hendrick Motorsports, the digital twin is already helping the racing team perform as a formidable competitor, despite some remaining obstacles. The plan calls for the digital master to encompass mechanical structures, the kinematics of the vehicle, the sheet metal placement, the duct work and the bracing of the cars—all created in the digital world first before building any physical prototypes. Not only does the approach enable more design freedom, Wall says, but it also delivers cost savings because the team only creates physical prototypes of optimal designs.
The digital twin’s ability to connect the virtual and physical worlds is also strategic to Hendrick Motorsports’ winning performance on the track. The sensored cars and test equipment produce a tremendous amount of data that can be mined for insights to fuel quick design adjustments in response to problems that occur or the ongoing rule changes.
“The biggest thing for us is to keep the process as nimble as possible,” Wall says. “The digital twin affords us a speedy response to make competitive changes, fix problems and create more performance at the track in a very tight window of time.”
More Info
Subscribe to our FREE magazine,
FREE email newsletters or both!Latest News
About the Author
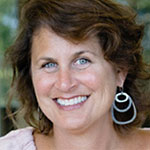
Beth Stackpole is a contributing editor to Digital Engineering. Send e-mail about this article to [email protected].
Follow DE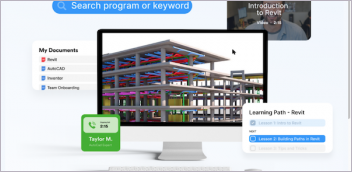
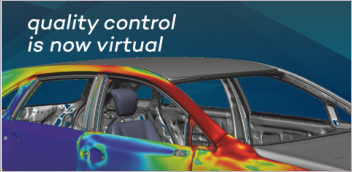
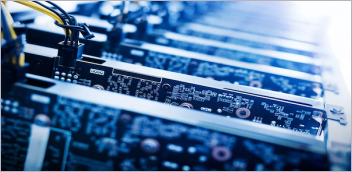
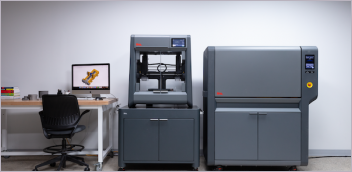