Latest News
March 25, 2013
By Mehrdad Zaneneh

Efficiency levels for many turbomachinery applications have matured in recent years, in part because of new regulations. The introduction of computational fluid dynamics (CFD) in the design process led to significant improvement in performance, but further improvements are increasingly more difficult to achieve. The conventional design process, which is based on iterative changes to geometry, relies heavily on previous design experience, and tends to encourage designers to stay within their comfort zones. This can limit design efficiency, making it more difficult to achieve significant breakthroughs or meet demanding multi-objective/multi-point/multi-disciplinary requirements of modern turbomachinery.
In principle, all turbomachinery applications share common challenges, whether one looks at multi-disciplinary problems, such as aerodynamic with mechanical performance, noise and efficiency in fans and compressors, or good suction performance. Robust design optimization must also be considered, the aim being to make the design performances less dependent on operating changes. Automatic optimization can help find solutions to all of these design challenges.
Weighing the Methods
The key in using optimization in design is to explore the design space as widely as possible. Gradient type methods are faster computationally, but tend to find local minima. The goal, then, is to use evolutionary type approaches such as a genetic algorithm (GA), which can explore the design space widely and find global minima of objective functions. GA also copes well with multi-objective criteria, and creates Pareto-optimal fronts that show trade-offs between different design parameters.
On the other hand, GA requires a large number of objective function evaluations—typically running into thousands. Because many problems are multi-point, this makes the application of GA, coupled with full CFD or finite element analysis (FEA) rather expensive computationally.
Another alternative is to use some form of surrogate model. In this approach, a design matrix is generated by using design of experiments (DOE), which is then used to create a surrogate model through a quadratic regression (response surface model) or other methods. These surrogates can then be validated—and, if accurate enough, GA can be run on them, saving computational time as each CFD or FEA evaluation becomes a polynomial evaluation. However, in general, error-free surrogate models can only be obtained with a handful of design parameters.
Enter 3D Inverse Design
The keys to making automatic optimization part of a commercial product development are 3D geometry parameterization and the number of input design parameters. One blade geometry parameterized by just 30 parameters requires close to 600 to 800 designs to be evaluated—just for the initial DOE database. Even with high computational capabilities, this is not something that allows for a design to be developed in a few weeks.
In contrast, parameterization of the same geometry via 3D inverse design methods can be done with six to 10 parameters. This means only 40 to 100 designs are needed.
So, how is blade geometry parameterized via 3D inverse design? Different approaches are used for blade geometry parameterization. In axial machines, it is customary to use some of form of surface definition. By contrast, in many radial and mixed flow applications, generally the blade angle is used to control the camber distribution. This is done using a number of control points between leading edge to trailing edge on 2D sections that are then stacked together to form a 3D geometry.
In 3D inverse design, users parameterize the blade loading, or pressure jump across the blade. The implementation is such that four to eight parameters of blade loading can cover as much design space as 30+ parameters required when using blade shape directly. This reduction in design parameters makes application of surrogate model a practical reality for 3D design.
When taking time and cost into consideration, 3D inverse design technology proves to provide the clear advantage in achieving turbomachinery efficiency and emissions reduction via automatic optimization. Examples have been widely published. In the pump industry, for example, Carver Pumps and Ebara Corp. have successfully applied this method. For axial and radial fans, papers and case studies are available for ebm-papst, Kitech and Daikin. Finally, McQuay presented the application of coupled 3D inverse design with DOE for optimization of centrifugal compressor stage for the refrigeration industry.
Mehrdad Zaneneh, Ph.D., is a professor of thermofluids at University College London, and founding director and CEO of Advanced Design Technology (ADT). Send e-mail about this article to [email protected].
Subscribe to our FREE magazine,
FREE email newsletters or both!Latest News
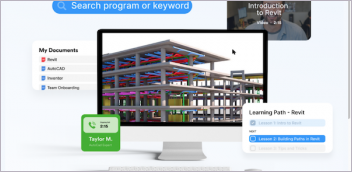
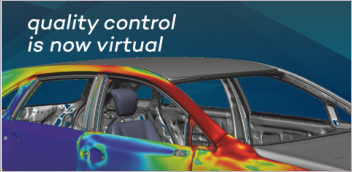
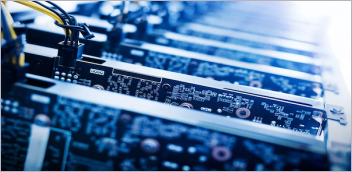
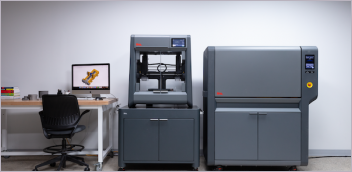