NVIDIA GTC 2016: The GPU Wants to Accelerate VR, AI and Big Data Analysis
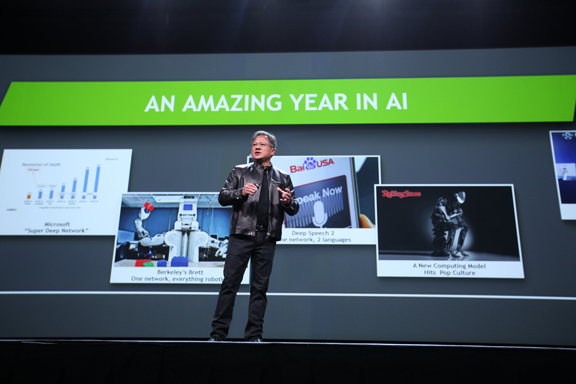
Once a game graphics company, NVIDIA now sets its sights much higher—on AI, VR, and Big Data. Company CEO Jen-Hsun Huang got ready to discuss advancements in AI at the GTC conference. (Image courtesy of NVIDIA)
Latest News
April 11, 2016
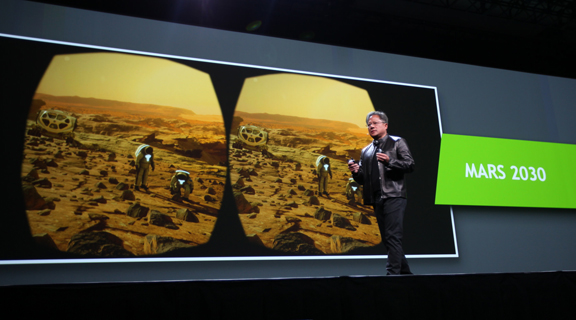
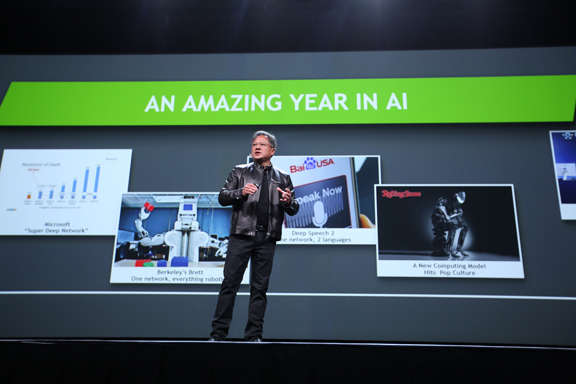
On Tuesday April 5, as he greeted the fans of GPU computing gathered inside San Jose’s McEnery Convention Center for the annual GPU Technology Conference (GTC), NVIDIA CEO Jen-Hsun Huang spoke of the new frontiers he’s setting his sight on.
Outlining the libraries available in the NVIDIA SDK, Huang discussed IndeX, a new Big Data visualization engine. “Running supercomputing generates terabytes and terabytes of data,” he said. “You now have to take that data and find insights from it. There’s simply no way to wade through that data on a spreadsheet. The only way is to visualize it. IndeX ix an in situ visualization platform, where you can simulate, generate the huge amount of data, and render the volumetric image in the exact same supercomputer. It’s the world’s largest visualization platform designed for visualizing supercomputing data.”
Later, discussing the newly released NVIDIA VRWorks, Huang said, “VR is an exciting new platform. With every new platform, you experience graphics in a different way. Because you’re seeing the graphics so close to you, and because it’s moving with your body and your head, any latency could cause you some amount of sickness. We created all kinds of new technologies for this platform. They’re incorporated into head-mounted displays.”
The debut of NVIDIA’s new hardware for AI developers came near the end of Huang’s keynote. He introduced the DGX-1, “the world’s first deep-learning supercomputer,” as he put it. It is the equivalent of 250 servers, he pointed out. “Just do some quick math,” Hung suggested. “250 servers at $10K a piece would come to 2.5 million ... Just the interconnects alone [for 250 servers] would be more than $500K. The DGX-1 is only $129,000.”
The technologies that were tried and tested in games laid the groundwork for the current exploratory initiatives in VR, AI, and Big Data. The physically realistic rolling terrains that shift in response to the player’s movements and the secondary characters with autonomous path-finding skills are but the beginnings of VR and AI. NVIDIA’s move to transform the GPU from a graphics engine to a computing platform is at the heart of its growth strategy.
Supercharged Computing
“The computer you need is not an ordinary computer. You need a supercharged form of computing. We call it GPU-accelerated computing,” said Huang. The company is now developing GPU architectures dedicated to AI and deep learning, he added.
This year’s GTC is the launchpad for the NVIDIA TESLA P100, “the most advanced hyperscale datacenter GPU ever built,” as Haung described it. He announced the new GPU is in production now. “The processor itself has over 15 billion transistors,” Huang pointed out. “It’s the largest chip that’s ever been made. It’s chips on top of stacks of chips, in the 3D stacking technology. Altogether, this chip has 150 billion transistors.”
The P100 comes with “new features, new architectures, and new instruction formats for Deep Learning [at] 16-bit performance,” Huang said.
Though its core business is graphics hardware, NVIDIA continues to build its software portfolio at an aggressive pace. In his keynote, Huang outlined the content of the NVIDIA SDK, which includes: ComputeWorks for training neural network training and big data visualization; DriveWorks for self-driving cars, VRWorks for virtual reality, GameWorks for videogame development, DesignWorks for photorealistic design visualization, and more. These software pieces serve as building blocks for GPU-accelerated tasks, aimed at dramatically speeding up research projects, engineering simulation, large-scale visualization, natural language processing, and AI.
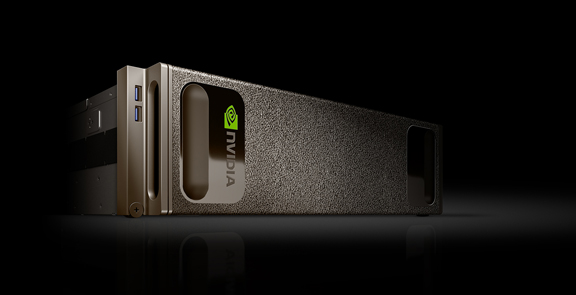
CAD to VR
One of the eccentricities of VR imagery processing rests with how human eyes perceive real panoramic imagery. Usually, the eyes focus on the center of the view, therefore objects in the peripheral vision of the warped view remain unfocused. By mimicking the same behavior in VR imagery (concentrated computing power to render the center, reduced resolution in the surroundings), NVIDIA’s GPU is able to deliver much better performance in rendering real-time VR content for head-mounted displays. (For more, read “Prelude to GTC: Are you Ready for the Era of Serious VR?”)
VR content is usually put together in game engines, such as Unity, Unreal, or CryENGINE. CAD users possess rich, detailed, VR-worthy content, but the stumbling block may be the CAD-to-VR hand-off—currently not a straightforward approach.
Michael Kaplan, NVIDIA’s global lead for media & entertainment, suggested, “CAD software makers might want to think about the hand-off process to the engine. Or, would they prefer to develop their own CAD-to-VR conversion software? After all, they’re software companies.” The latter, however, could prove more than what a typical CAD software developer could undertake, because, Kaplan pointed out, “Most of them have never written real-time VR software.”
Kaplan believes the manufacturing industry is fertile ground for VR. “Imagine putting on a head-mounted display and being able to experience a car that’s still in development. You’d be able to say, Let me see what this looks like in blue. Let me see this with a different rim. Let me try on a different kind of interior ...,” he said
The First Crack at DGX-1
In his keynote, Huang promised to give “the pioneers of AI” the first crack at NVIDIA’s supercomputer for AI. Researchers at UC Berkeley, Carnegie Mellon, Standford, Oxford, and NYU are among those who will receive “the first DGX-1 coming off the production line,” said Huang.
Jim McHugh, NVIDIA’s VP and GM, said, “After the AI researchers, data scientists are going to get the DGX-1, not just because their computational needs are intense, but also because they’re subject matter experts.”
If the $129,000 price tag for NVIDIA’s hardware is a steal (that is, for researchers working on AI), the price tag of TensorFlow, the AI-training software, is even cheaper. It’s Open Source—free. The optional CUDA installation in TensorFlow setup gives you GPU acceleration in using TensorFlow.
The DGX-1 is essentially a supercomputer assembled out of eight NVIDIA TESLA GPUs, preintegrated with NVIDIA’s Digits software for deep learning and job management software. “You can download a lot of these components and try to put together an AI-training machine,” said McHugh, “but you won’t get the same performance like the DGX-1 because we tuned it.”
In this early stage, most AI-powering hardware still remains too costly for ordinary users. However, it’s not far-fetch to imagine that, in the not-so-distant future, some cloud-hosted AI-training platform providers might emerge. Under the on-demand model, someone could upload a sample dataset (say, shapes of different road signs in multiple countries) to train a self-navigation system for an autonomous car.
The current use of VR in design and manufacturing is confined to presentation primarily, to offer customers and clients a chance to experience a product while it’s still in the concept phase. And the current use of AI in the same sector is fairly limited, with autonomous cars at the forefront. But stunning breakthroughs are inevitable when VR, AI, and Big Data converge.
Subscribe to our FREE magazine,
FREE email newsletters or both!Latest News
About the Author

Kenneth Wong is Digital Engineering’s resident blogger and senior editor. Email him at [email protected] or share your thoughts on this article at digitaleng.news/facebook.
Follow DE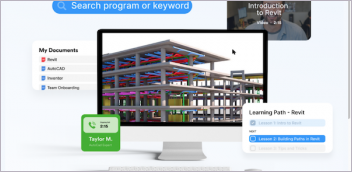
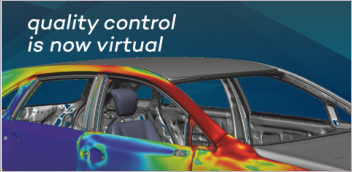
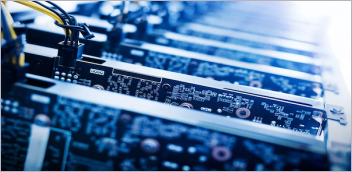
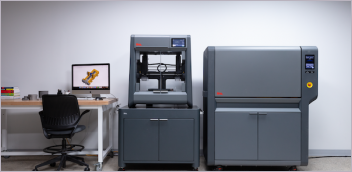