
In its briefing slides for the ISC show, CPU maker Intel claims CPU’s superiority over the GPU.
Latest News
August 18, 2016

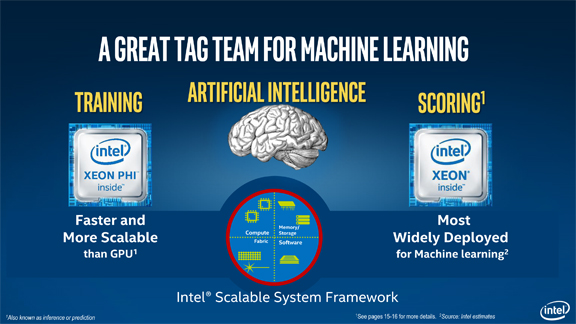
In June, when Intel began briefing the press about its upcoming announcements at the ISC (International Super Computing) Conference, the company circulated a presentation deck titled “Fuel Your Insight.” In one slide, the company claimed its Intel Phi processor is “faster and more scalable than the GPU” in machine learning. To back this up, it cited the following comparison:
Up to 2.3x faster training per system claim based on AlexNet topology workload (batch size = 1024) using a large image database running 4-nodes Intel Xeon Phi processor 7250 (16 GB MCDRAM, 1.4 GHz, 68 Cores) in Intel® Server System LADMP2312KXXX41, 96GB DDR4-2400 MHz, quad cluster mode, MCDRAM flat memory mode, Red Hat Enterprise Linux 6.7 (Santiago), 1.0 TB SATA drive WD1003FZEX-00MK2A0 System Disk, running Intel® Optimized DNN Framework, Intel Optimized Caffe(internal development version) training 1.33 billion images in 10.5 hours compared to 1-node host with four NVIDIA Maxwell GPUs training 1.33 billion images in 25 hours (source: http://www.slideshare.net/NVIDIA/gtc-2016-opening-keynoteslide 32).
The GPU maker NVIDIA took issues with this claim, publishing its own retort in a blog post titled “Correcting Intel’s Deep Learning Benchmark Mistakes.”
The post’s author Ian Buck, NVIDIA’s VP of the Accelerated Computing business unit, wrote, “Intel used Caffe AlexNet data that is 18 months old, comparing a system with four Maxwell GPUs to four Xeon Phi servers. With the more recent implementation of Caffe AlexNet, publicly available here, Intel would have discovered that the same system with four Maxwell GPUs delivers 30% faster training time than four Xeon Phi servers.”
He went on to point out, “In fact, a system with four Pascal-based NVIDIA TITAN X GPUs trains 90% faster and a single NVIDIA DGX-1 is over 5x faster than four Xeon Phi servers.”
Pascal is NVIDIA’s latest GPU architecture, introduced in April 2016. It’s a successor to Maxwell, introduced in 2014. The ISC Conference marked the launch of the second-generation Intel Phi processors.
CPU maker Intel and GPU maker NVIDIA compete for market dominance in high performance computing (HPC). Intel targets this market with its Intel Phi product line; NVIDIA pursues the same market with its Tesla product line.
Machine learning—the use of supercomputers to process and analyze large data sets—is seen by processor makers as an attractive market, because it’s expected to drive up the sales of large-scale systems powered by CPUs or GPUs. Opportunities in this area could offset the slumping sales of personal computers, resulting in part from the rise of virtualization and mobile computing.
To dispute Phi’s superior scalability stated by Intel, NVIDIA wrote:
Intel is comparing Caffe GoogleNet training performance on 32 Xeon Phi servers to 32 servers from Oak Ridge National Laboratory’s Titan supercomputer. Titan uses four-year-old GPUs (Tesla K20X) and an interconnect technology inherited from the prior Jaguar supercomputer. Xeon Phi results were based on recent interconnect technology. Using more recent Maxwell GPUs and interconnect, Baidu has shown that their speech training workload scales almost linearly up to 128 GPUs.
Baidu is a large web services company based in China, where it is the country’s top search engine. The exchange reveals the importance of interconnects—something often overlooked—in the performance of large-scale systems. In such systems, hundreds of thousands of processors attack a computing job in a coordinated fashion. Therefore, the bottlenecks in the interconnects could compromise the capacity of the underlying processors, whether they’re CPUs or GPUs.
“It is completely understandable that NVIDIA is concerned about us in this space,” an Intel spokesperson replied via email when asked to comment on NVIDIA’s blog post. “We routinely publish performance claims based on publicly available solutions at the time, and we stand by our data.
Subscribe to our FREE magazine,
FREE email newsletters or both!Latest News
About the Author

Kenneth Wong is Digital Engineering’s resident blogger and senior editor. Email him at [email protected] or share your thoughts on this article at digitaleng.news/facebook.
Follow DE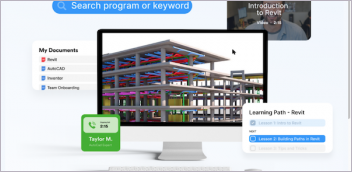
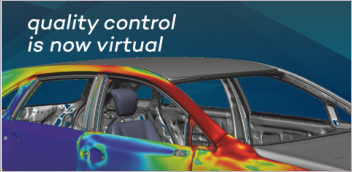
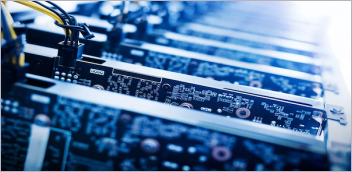
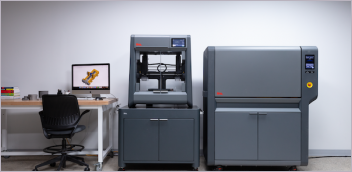