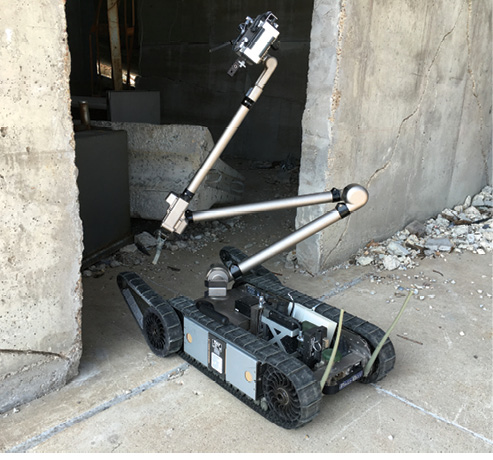
Sensor-laden robots, like the iRobot PackBot, can go places too dangerous for humans, such as the Fukushima nuclear reactor. Image: CRASAR
Latest in High–performance Computing HPC
High–performance Computing HPC Resources


Latest News
May 24, 2017
When Hurricane Katrina devastated the Gulf Coast in 2005, it was not only the costliest and one of the deadliest natural disasters in the history of the United States, it also marked the first time drones were deployed during a disaster.
Using small, unmanned aerial vehicles (UAVs), the Safety Security Rescue Research Center (SSRRC) was able to help survey the Pearlington, MS, area for stranded survivors when all the roads into town were blocked. With video footage from the drones, emergency responders were able to determine that no one was trapped, and that floodwaters from a nearby river would not create any additional safety issues.

There have been significant advances in the technology used in disaster response since then, thanks to a combination of advanced robots and drones, social media and artificial intelligence (AI). Using drones equipped with cameras and sensors, relief agencies and first responders can safely survey affected areas, search for survivors, measure flood water levels, test for radiation, examine critical infrastructure (like power plants or dams) without endangering employees, and potentially even deliver some supplies.
“There’s really been a shift since 2010 as less-expensive quadcopters and other aerial devices have come out,” says Dr. Robin Murphy, professor of computer science and engineering at Texas A&M University and director of the Center for Robot-Assisted Search and Rescue (CRASAR), which is one of only two centers in the world dedicated to disaster robotics. “It’s not really the robot that’s interesting anymore, but it’s the data that you use.”
It’s not really the robot that’s interesting anymore, but it’s the data that you use.
Additionally, computer vision and machine learning have helped enable new ways to obtain and analyze high-resolution imagery. “Using real-time images from UAVs, you can start doing orthomosaics, tie them together in a master image and get a 3D digital surface map that is pretty accurate,” says Murphy, who was also on the team that deployed the drones after Hurricane Katrina.
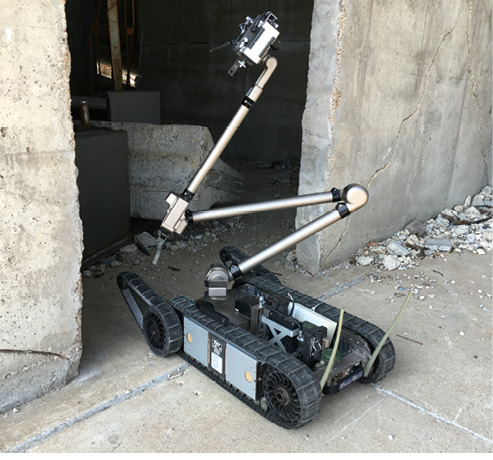
Even with these advancements, getting the right data to the right people on the ground in a timely fashion is still the main challenge in disaster response and relief efforts. “You have to reduce friction and make reliable information available to people on the ground,” says Steve Schwartz, social impact marketing manager at Tableau Software, which makes business intelligence and analytics systems. “In the minutes and hours after a disaster, there is asymmetry of information. As much as we can do to give responders a clear picture of what’s going on, that’s really our priority.”
As an example, Tableau has worked with NetHope, which links nonprofits with technology companies, on a number of projects. In one instance, they collected various types of maps in Ecuador to create a single repository so that responding agencies could quickly locate useful maps and related information without lengthy searches.
Data Helps Disaster Planning, Response
Different organizations are leveraging machine learning and analytics in different ways.

In 2012, the American Red Cross and Dell launched a Digital Operations Center, which the companies described as the first social media-based operation for humanitarian relief. The center uses Dell technology and consulting services to help engage the public during emergencies.
The Red Cross tested the system during a spate of Midwestern tornadoes, and team members were able to determine where to position workers on the ground using the technology. The system can help the Red Cross obtain additional information from affected areas during emergencies, anticipate the public’s needs and connect people with resources like food, water, and shelter.
The Red Cross also teamed up with Facebook for its Missing Maps project to help better map people in areas at high risk of natural disasters or emergencies. Facebook uses satellite imagery and computer vision to create detailed population density estimates, and that data can be used to more efficiently map at-risk communities. The Red Cross also debuted a new system to help predict and prepare for flood risks this year to help forecast flood relief funding needs. This forecast-based financing system is being implemented at the Nangbéto Dam in Togo, Africa. Hydropower operators in the region are using machine learning to predict flood risks and communicate them to nearby communities.

The organization has studied the use of drones to improve disaster response and relief efforts. However, in the U.S. the commercial use of drones is limited to those with exemptions granted by the Federal Aviation Administration. Certain regulatory and privacy issues remain unclear when it comes to drone use.
Facebook has been leveraging its own network and analysis tools for disaster response as well. During the mass shooting at the Pulse nightclub in Orlando, for example, the site’s Safety Check function helped people keep track of friends and loved ones in the area. The company plans to expand the service into a full-blown crisis hub that can help people coordinate response activities and watch live video feeds. The company’s algorithms can automatically establish a news hub for any given crisis based on posts and mentions, along with data from emergency services.
Some of the most dramatic uses of technology are those that combine UAVs with computer vision and AI or machine learning. CRASAR’s Murphy has been working with disaster response robotics for decades. She helped deploy robots to investigate the World Trade Center site after its collapse in a terrorist attack in 2001 and helped send similar aerial drones to the Fukushima nuclear reactor to test radiation levels.
CRASAR is able to test out robotic systems at Disaster City, a 52-acre reconstruction site and training facility at the university. The CRASAR team not only participates in actual disaster robotics deployments around the globe, but also helps test these systems in real-world simulations. For example, CRASAR and Roboticists Without Borders took part in a four-county wilderness search-and-rescue exercise in 2017 using UAVs and a small, unmanned marine vehicle to identify and recover a submerged body.
But there are still obstacles. During the Oso, WA, mudslides in 2014 Murphy’s team and other drone companies arrived to help map the disaster site and help locate survivors, but they weren’t allowed to take off because of privacy concerns and strict local laws governing drone usage.
A New View of Visual Data
UAVs armed with cameras can generate hundreds of images in one 20-minute flight, which can bog down responders trying to evaluate the data. During 2015 flooding in Texas, Murphy had her students develop applications to improve and automate the process of evaluating those photos.
“The low-hanging fruit in this is finding anomalies in the images that look like a sign of a person or man-made debris,” Murphy says. “Computer vision doesn’t make this perfect, but you can triage the images and sort them, and the program can circle things for the human operators to look at. You can take a few hundred images that would have taken a day to get through, and get that down to 90 minutes.”
Using computer vision and machine learning, they created programs that could identify debris and highlight areas where people also might have been swept up in the flood. They were able to leverage deep learning research from the University of Maryland to help detect anomalies in the photos and spot debris piles that were big enough to hide a body. The system isn’t perfect, but it helps responders better identify areas that warrant further investigation much faster and more accurately than a manual search.
That ability to create 3D models using images from drones can revolutionize the way aerial search and rescue works. “This was a laboratory curiosity 10 years ago, but now you can build these 3D models on the fly using video collected from a drone,” says Larry Davis, professor at the Institute for Advanced Computer Studies at the University of Maryland, who has helped lead the computer vision and deep learning research. “You can then use computer vision techniques for material classification or to identify areas where you want to get better images.”
According to Davis, deep learning advancements have really revolutionized how computer vision and machine learning can be used for this type of image analysis.
The EU’s Joint Research Centre (JRC) and satellite imaging company DigitalGlobe also are developing an algorithm to help interpret images for disaster response. Another company, One Concern, is using machine learning and analytical disaster assessment to help emergency operations centers get instant recommendations on response priorities and resource utilization.
Kashmir native Ahmad Wan founded the company after seeing the disorganized response to rescue during flooding there in 2014, as well as during an earthquake in Napa County, CA, when he returned to his studies in Stanford that same year.
He and his partners created a machine learning model that could predict structural damage to buildings with a high degree of accuracy. The solution can generate a color-coded map of areas with likely structural damage that authorities can use to help guide their response. It also can provide demographic data to help prioritize responses as well.
In Massachusetts, utility provider National Grid partnered with Massachusetts Institute of Technology to build a statistical model to predict localized utility interruption patterns. The machine learning predictive algorithm considers the physical properties of the network, historical weather data and environmental information to help predict outages and damage.
Utilizing artificial intelligence and data analysis is already making a difference. The Netherlands Red Cross developed an initiative in the Philippines called 510 to help coordinate aid response during typhoons. Using weather information, data from past disasters, rainfall amounts and other information, the system creates a Priority Index to identify where the most affected areas will be. In 2016, the system helped predict, with a high degree of accuracy, the most affected communities during Typhoon Haima. That helped the Red Cross deploy aid resources much faster.
Better Data Sharing
Schwartz says Tableau has been involved in a number of projects that leverage data analysis and sharing to enhance response activities. The company has been working with the United Nations Office for the Coordination of Humanitarian Affairs (OCHA), for example, to help establish pre-staged data sharing agreements in advance of crises so that disparate agencies and governments can quickly pool resources during a disaster instead of haggling over data sharing protocols that can delay activities for days or weeks.
During the 2014 Ebola outbreak in West Africa, Massachusetts-based social enterprise firm Dimagi used Tableau Server and Desktop as part of its CommCare informatics system to collect data on ebola transmissions. They were able to provide information on contacts infected patients had made to health ministers to help map out where resources might be needed.
The UN World Food Programme (WFP) is also using Tableau’s solution for vulnerability analysis and mapping activities in Nigeria. The WFP was able to obtain data on food prices and availability via mobile phones even in areas that were inaccessible because of the Boko Haram insurgency. Data can be presented in easily understandable tables and graphics to help gauge food security and develop response plans.
Next Phases
CRASAR’s goal is make sure local responders are able to afford and support disaster robotics systems and computer vision tools without having to borrow them from specialized agencies or the federal government. “You can’t wait two days for someone to fly it in,” Murphy says. “If you are the local emergency manager for a county, you can grab these assets and get the data to the transportation people and emergency managers.”
Murphy says autonomous marine vehicles could be the next frontier of disaster response robotics. CRASAR has run tests of SARbots, marine robots with sensing capabilities that can use sonar to help inspect underwater damage.
“Nobody pays attention to marine-based robots,” Murphy says. “A lot of critical infrastructure winds up under water in these events. UAVs have really captured everyone’s attention, but you need to take a land, sea and air approach.”
As computer vision technology continues to improve, driven in part by investments from automotive companies developing self-driving cars, Davis expects adoption to increase. “There’s also going to be more activity over the next five years in satellite image analysis because of improvements in the quality of sensors used by satellites,” he says.
In the long run, the industry will work toward decreasing the cost of these systems so they can work on mobile devices. “There is a lot of work going on in trying to scale down the deep learning system so you can put them on a mobile device and give them to a large number of people,” Davis says.
Combined with low-cost UAVs and robots, that could revolutionize the effectiveness of local first responders and relief agencies.
For More Info
American Red Cross Digital Operations Center
Center for Robot-Assisted Search and Rescue
Institute for Advanced Computer Studies at UMD
Subscribe to our FREE magazine,
FREE email newsletters or both!Latest News
About the Author
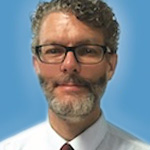
Brian Albright is the editorial director of Digital Engineering. Contact him at [email protected].
Follow DE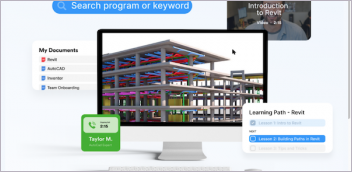
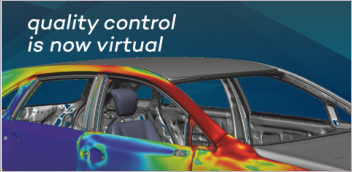
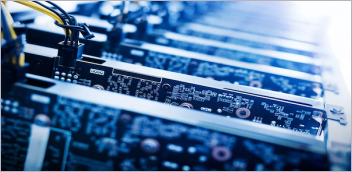
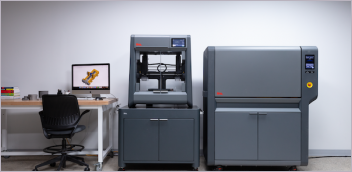