Latest News
October 20, 2023
As artificial intelligence marches on to permeate many different industrial sectors, the automotive industry is one that stands to significantly benefit from this advancement. The auto industry represents about 3% of America's GDP. In 2022, the industry sold an estimated 13.75 million cars and light truck vehicles.
MathWorks is a software solutions provider to the engineering efforts of so many industries. Seth DeLand is the AI Product Marketing Manager at MathWorks in Novi, MI. We spoke to Seth to better understand his perspective on the application of AI in the automotive industry.
Digital Engineering: What is your perspective on how AI for Simulation is impacting automotive manufacturing?
Seth DeLand: As the automotive manufacturing process has become more complex, simulation has played an increasing role in optimization and troubleshooting issues before they manifest on the factory floor. At the same time, recent advances in AI have made it possible to apply AI in new use-cases that historically were too challenging to automate or uneconomical to solve.
Recently, MathWorks has worked with a handful of automotive manufacturers on applying AI to audio recordings taken during the manufacturing process. They are using this audio to characterize the health of machinery, and to characterize the quality of the part being produced. By using historical audio data with known condition indicators, they can train AI models that accurately predict the health of the equipment or quality of the part. For example, they train a model using audio signals to identify degradation in manufacturing tools such as dies. Simulation comes into the picture when it is time to understand how the AI model will perform when it interacts with other parts of the application, such as the microphone, audio filtering, or other parts of the data processing pipeline. By simulating the system, including the AI model, engineers can validate that the application will perform as expected before deploying it to devices in the field.
Additionally, equipment manufacturers are increasingly looking at new AI-based controls technologies like reinforcement learning that rely heavily on simulation during the development process. In reinforcement learning, the computer learns the control strategy by running thousands of simulations and tweaking the controller based on the simulation result. This enables the computer to learn how to make decisions without human intervention and can potentially solve challenging problems in robotics and scheduling.
Digital Engineering: How is design software and modeling and simulation software influencing automotive manufacturing and/or enhancing the industry's manufacturing digitization?
Seth DeLand: Engineering software like MATLAB and Simulink are helping engineers bring AI to the manufacturing process. Much of the research in AI is being done in areas like large language models and image classification. It can be challenging to see the connection between this research and applications within manufacturing. MathWorks focus has been to take the tools coming out of the research and make them suitable for engineers who don’t have the time to sift through the research. By doing that, MathWorks enables engineers to apply AI in new and novel ways such as audio classification for predictive maintenance, and new applications of visual inspection in the manufacturing process.
Digital Engineering: What are some of the challenges in auto manufacturing that engineering tools such as simulation can solve? ie. less waste, more efficiencies in production output, less risk of downtime, etc.?
Seth DeLand: Predictive maintenance continues to be an application where AI and simulation are seeing success. Simulation can come into play at multiple stages of the process when developing a predictive maintenance algorithm. It can be used for synthesizing failure data when a lack of recorded data is available to train the AI model, and simulation can be used to verify that the predictive maintenance algorithm works correctly when integrated with the other applications. The end result is less risk of downtime, and increased efficiencies from better-performing machinery.
AI and simulation can also play an important role in developing a defect detection algorithm. Such algorithms may be developed using images from a camera, audio from a microphone, or other signals from sensors on the machinery. In any case, simulating the performance of the AI-based defect detector can help understand the detectors abilities to identify defects and improve the quality of parts.
More MathWorks Coverage
Subscribe to our FREE magazine,
FREE email newsletters or both!Latest News
About the Author
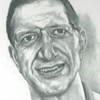
Jim Romeo is a freelance writer based in Chesapeake, VA. Send e-mail about this article to [email protected].
Follow DE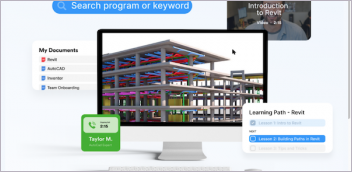
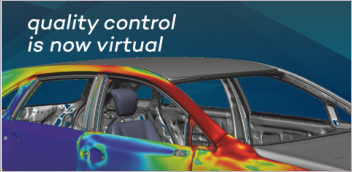
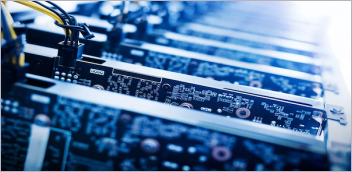
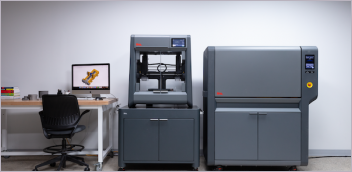