Artificial Intelligence Wants a Seat at the Design Table
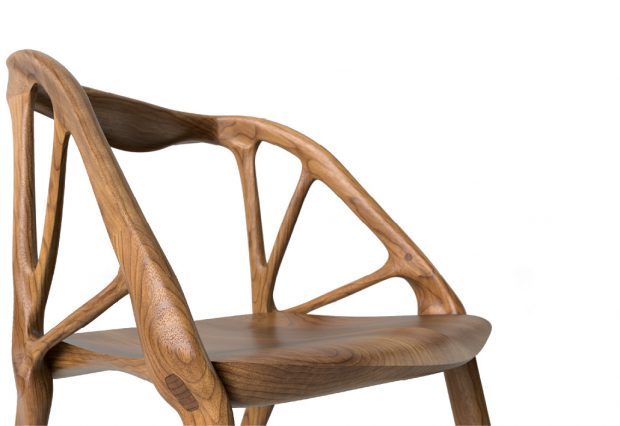
Rendered views of the Elbo Chair, designed in Dreamcatcher using algorithms. Image courtesy of Autodesk.
Latest News
December 1, 2017
We all know what a chair looks like; but how do you teach a computer what a chair is supposed to look like? That was one of the puzzles Arthur Harsuvanakit had to solve to design an ergonomic chair using Autodesk Dreamcatcher, a generative design software package.

“I gave the software the absolute minimal input on what a chair is supposed to be,” explains Harsuvanakit. “[For the seating area,] I gave the software an elevated platform as input, then asked for all the different options to support it.”
That was the digital genesis of what would later become the Elbo Chair, produced at Autodesk’s Pier 9 Workshop in San Francisco. The name came from the Danish Furniture Master Hans J. Wegner’s Elbow Chair, which served as an inspiration for this project.
When using a parametric 3D CAD modeling program, the design engineer typically uses his or her judgment, intuition and industry experience to come up with a concept, then digitally sculpt the shape in the software. Generative design or algorithm-driven design turns this process on its head.
Instead of modeling a preconceived idea in the software, the designer provides the generative design software with requirements, such as where the object needs to withstand a load, what load or pressure is expected, what material choices are acceptable and so on. The software then mathematically computes and recommends the best topologies or shapes based on these specifications. For some, the new paradigm is AI-assisted design.
Generative design tools and algorithm-driven design projects are growing. More and more software vendors are now looking to incorporate AI-like features into their offerings. For both the designers and software developers, a new challenge has appeared on the horizon. How do you reframe subjective human preferences, such as comfort and aesthetics, as computable design parameters?
Getting Comfortable with AI
A senior designer in Autodesk’s Office of the CTO, Harsuvanakit had tinkered with algorithm-driven designs before. The small brackets and desk lamps he designed using Dreamcatcher were his warm-up acts. “The software gives you a lot more options—some of them you might not have even thought of on your own. As a designer, you learn to curate these forms, [and] narrow them down by adding more constraints,” he notes.
Now, smaller objects with odd geometric angles that might have been impossible to machine could easily be produced with 3D printers. But a chair is of considerable size, larger than the build chamber of most 3D printers. To further reduce his design options for the Elbo Chair, Harsuvanakit used material and manufacturing requirements as guidance. His choice of material was wood, and fabrication was CNC. New forms generated by Dreamcatcher with the additional inputs were much more feasible for machining.
41% of DE survey respondents chose AI and machine learning as having the biggest impact on product design and development over the next 5 years.
“The initial exploration [for supporting a flat seating area] resulted in about 300 options,” Harsuvanakit recalls. “Once we gave the software more loads, directions and obstacles, we got to about 200 options. The majority of these options were early iterations on a timeline to a more mature generation. Each iteration is a slightly different form, and in the process, you sometimes don’t pick the most mature generation. There were about 60 real variation differences for the initial exploration, and for the final setups we considered about five options, with about 120 iterations behind each option.”
Recounting the project, Autodesk blogger Scott Sheppard wrote: “Compared to the original design, Dreamcatcher’s solution has 18% less volume and decreases the max displacement by 90.4% as well as decreases the max von Mises stress by 78.6%” (It’s Alive in the Lab, Sept. 14, 2016, labs.blogs.com/its_alive_in_the_lab).
The Elbo Chair will be on display at the SFMOMA from January to May 2018, as part of its “Designed in California” exhibit. Dreamcatcher began as a generative design software research project, but is now a commercial product. Autodesk Generative Design is currently available to Netfabb Ultimate subscribers with wider rollout already scheduled for the near future.
Math’s Surprising Answers
Math can surprise even an experienced designer like Harsuvanakit. Some support options the software considers to be mathematically optimal were “not the most obvious ones to me,” he says. “That’s why the method challenges your understanding of structures; it challenges your traditional views of form,” he added.
“AI is already a part of our development. We strongly believe that AI will help lead development in the future.”— DE survey respondent
“A lot of times, the topology optimization software gives you forms that are not ready to use,” says Himanshu Iyer, product marketing, Siemens PLM Software. “They’re still faceted models, and the designer needs to bring them into the CAD system for rework. That means fixing surfaces, adding fillets and blends, and so on. NX with Convergent Modeling technology is the only platform that can fully utilize the facet models created by topology optimization.”

In one in-house project, Iyer recalls, Siemens PLM software used HEEDS multidisciplinary design exploration software to develop an automotive component. “We ran 400 design iterations in eight hours, using HEEDS and NX’s topology optimization. The design the software came up with would have been very difficult even for an experienced designer to come up with,” notes Iyer.
Tribal Knowledge
“AI is not the wave of the future. It’s already here. So we need to figure out how to ride that wave,” says Harry Zhong, Stanley Black & Decker’s innovation technical lead for infrastructure.
One software Zhong and his team use is Generate from Frustum Inc., a generative design program that offers topology optimization. “Because of our application, we need the strength and durability of metal. But metal parts in infrastructure projects tend to be heavy,” he says. With Generate, Zhong and his team can look at ways to redesign some parts as lighter versions that can withstand the same loads and pressures.

“The software is ultimately an FEA (finite element analysis) tool, so if you set it up correctly to mimic the real-world scenario, the software gives you pretty good results,” says Zhong. “In the times when I ended up with outlandish, impossible geometry, it was because I didn’t set up the problem correctly, to define the contacts as planar, bolted or fixed.”
Luke Ice and his colleagues from the 3D Printing Group of DePuy Synthes, a Johnson & Johnson company, operate as the company’s in-house support unit for additive manufacturing (AM) projects. Ice is also evaluating Generate as one of the AM-friendly design tools.
“AI and machine learning will likely be used to build more powerful simulation platforms. It is unlikely to have a short-term impact on our development process in the near future.”— DE survey respondent
“A lot of conventional manufacturing rules of thumb are based on the idea that you start with a block of material, then you cut away to get to what you need,” says Ice. “It takes a different perspective to design for AM. Traditionally, you drill a hole to reduce weight. But with AM, you can actually make that hole aesthetically appealing with patterns.”
With medical and surgical instruments, the look, grip and comfort matter just as much as the structure’s load-bearing capability. Ice and his team have intimate knowledge about how surgeons and doctors work, down to their preferred postures in the operating room. They also know the gray areas where surgeons don’t have a clear consensus. This knowledge, Ice points out, is what he uses when evaluating the forms generated by the software.
“Having a generative design tool is like having hundreds of interns who can churn out design iterations for you,” says Ice. “But you still need your tribal knowledge to evaluate them. Sometimes, what’s mathematically optimal is a shape that I know we wouldn’t want to put our company logo on.”
Manufacturing Constraints
A topology optimization program can compute loads and offer optimal shapes, but it doesn’t necessarily understand manufacturing constraints—not unless it’s written into the form-generation logic. “Often, what the software recommends is not easy or possible to fabricate,” says Harsuvanakit.
Autodesk’s portfolio includes software like Moldflow, for simulating injection molding, and Inventor HSM, for simulating CNC operations. Incorporating nuggets of these underlying technologies into generative design algorithms could bolster the software’s ability to recognize design options unsuitable for certain production methods and materials.
“Coding manufacturing constraints is an area of research for Autodesk,” says Harsuvanakit. “In a way, it’s an easier problem to solve.” What are much harder to program or distill into a series of equations are the subjective criteria, such as aesthetics, ergonomics, novelty and comfort.
“In the newest release, NX 12, the manufacturing constraints are already built into the topology optimization algorithm. It means the designer will have fewer steps to take to make the design manufacturing-ready,” says Siemens’ Iyer.
“Frustum’s Generate has built-in manufacturing constraints,” says Black & Decker’s Zhong. “Even if I’m a good CAD designer and can model the shape I want, there are always those odd draft angles that won’t work in machining or an area that proves too thick to produce.”
In theory, generative design software with built-in manufacturing rules can prevent or reduce these artifacts in the form-generation phase. Thus, it goes a long way to reduce the designer’s burden in form assessment and manual model refinement.
Generative Speak
If parametric CAD modeling is a distinct skill, so too is generative modeling. Whereas parametric modeling depends on knowing how to use lines, circles and arcs to produce the desired geometric solids, generative modeling requires the ability to translate design ideas into computable parameters.
“Generative design is the next generation of design software, as we see it,” says Iyer. “But the first step is still the designer providing the best input. Otherwise, as the saying goes, garbage in, garbage out.”
“The challenge for the designer is to turn what he or she wants into a set of requirements,” says Dr. Uwe Schramm, Altair CTO for solvers, optimization and smart multiphysics solutions. “We used to come up with the design, then use simulation to test the concept. Now, we use simulation, topology optimization and machine learning to come up with the design. [The machine’s answer to the problem] can be completely different from what you guess it might be.”
Altair’s OptiStruct solver is the engine behind its popular topology optimization software solidThinking Inspire. The company also offers HyperStudy to run design of experiments (DOE) studies. The software can be deployed for approximation, optimization and stochastic studies. Schramm says, “The need for manufacturing constraints has been recognized by early adopters in the 1990s. OptiStruct released minimum member-size control, draw direction and other manufacturing constraints in the late 1990s, early 2000s.”
Solver Training
MSC Software’s simulation products include an estimator, which you can use to obtain the approximate memory, computing cores and time needed to run simulation. Horen Kuecuekyan, MSC Software’s head of AI, reveals that the latest version of the estimator is powered by pretrained optimization algorithms derived from machine learning, a data-driven approach to develop automation algorithms.
“The basic requirement of machine learning is a significant amount of data to train the [mathematical] model,” says Kuecuekyan. “Take simulation runs on software like MSC Adams [software for multibody dynamics simulation]. If you run simulation on pretrained data, productivity and performance go up.”
Fewer parameters lead to faster processing times. But when working with dozens of parameters to generate hundreds of design alternatives, understanding each parameter’s effect on the output design is sometimes beyond human comprehension. In the future, Kuecuekyan believes design software will allow users to apply machine learning to identify the most critical parameters in design of experiments (DOE) studies. Such a tool is “a work in progress” at MSC, according to Kuecuekyan.
The machine learning team headed by Kuecuekyan includes data scientists, among others. “Our goal [is] to include a machine learning component in each and every MSC product,” he says.
New Design Thinking
Schramm believes AI-driven design will usher in a rich knowledge repertoire for design, available for query. “Twenty or 30 years ago, we were talking about knowledge-based engineering,” he says. “But now, knowledge-based engineering may come on top of a neural network that helps you identify your design answers based on certain keywords. Some of these technologies will find their ways into modeling tools like solidThinking Inspire.”
The workflow Schramm suggests is closely linked to the cloud. So is the simultaneous exploration and validation of hundreds or thousands of design iterations. Neither is well-suited for an isolated desktop computing environment with finite computing power. Both point to the inevitable conclusion that design software must evolve from the present desktop-centric model to the cloud-hugging model. It doesn’t necessarily need to become browser-based software (though some like Onshape have made that choice). But it would need to offer the option to reach out for additional data or computing power.
“The math and physics of displacements and loads don’t change,” notes Kuecuekyan. “But with machine learning, the software may be able to predict how to get similar results without having to even run the solver.”
“[An algorithm-driven approach] forces you to think of the big pictures, like how the product will be secured, where it will face a lot of pressure or weight. That leads me to wonder if the part really needs to be symmetrical, for example,” says Zhong.
“The role of the designer is to understand the problem they’re trying to solve, and translate that into constraints in the generative objectives,” says Harsuvanakit. “The AI is your collaborative partner.”
More Info
Subscribe to our FREE magazine,
FREE email newsletters or both!Latest News
About the Author

Kenneth Wong is Digital Engineering’s resident blogger and senior editor. Email him at [email protected] or share your thoughts on this article at digitaleng.news/facebook.
Follow DE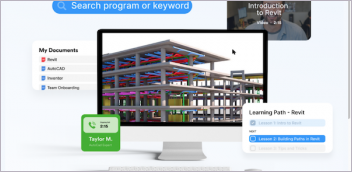
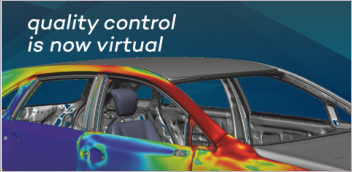
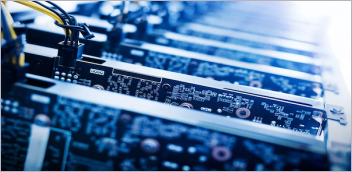
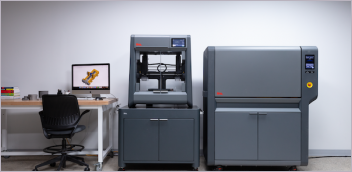