
Fig. 1: Harnessing AI, autonomous vehicle development teams can use physically accurate simulation and 3D environments to create, test and validate designs for AV systems and services. Image courtesy of NVIDIA.
Latest News
June 8, 2023
An actualized reality of autonomous vehicles (AVs) safely and efficiently delivering people and goods to their destinations depends largely on how carmakers harness the power of artificial intelligence (AI). AI’s influence already spans the entire spectrum of the AV’s lifecycle—pushing the limits of design, functionality and performance on the road—but the industry still has far to go before it can extract the full benefit of AI and achieve full driving automation.
The increasing number of sensory inputs available to AVs offers the prospect of a more accurate and detailed understanding of the operating environment, but this data still does not provide the visibility needed to meet safety requirements. Coming to terms with the complexity of the application and filling gaps in the picture of the physical forces at play will be the next benchmark for AV advances.
To understand just how close the idea of AV is to becoming a reality, it’s important to appreciate where the power of AI is being brought to bear and how effective current technology really is. To do this, let’s begin by examining AI’s impact on the fundamental tools and practices of the design process (Fig. 1).
Expanding the Data Foundation
The fundamental mechanism of the AI systems driving AV technology is the algorithm. Algorithm creation begins with the collection of huge amounts of high-quality, labeled physical data. This data is used to train, test and validate the algorithms.
“To achieve robust and reliable AI systems, it is important that the training data accurately represents the richness and diversity of the real world,” says Ben Alpert, director of engineering at Nauto. “For example, the dataset should represent different environment conditions, including things like low light or glare.”
The good news is that the industry now has a number of AI software development tools that collect, label, index, archive and manage the data needed to train AV algorithms. Current technology labels not only include objects within captured images and video frames, but also scenarios and conditions in video sequences.
Furthermore, the data collection conditions are also labeled. This provides a matrix of conditions that engineers can use to test the performance of algorithms against a wide range of scenarios, weather conditions and times of day. When performance doesn’t meet key performance indicators, the engineers collect and process more data for validation.
After data is processed, AI engineers use the labeled data to train algorithms for perception and other self-driving functions. This is an iterative process in which the engineers adjust algorithm parameters as needed and then retrain the algorithms, at which point the next set of labeled data is added to the training set. This process continues until the desired model performance and accuracy are achieved.
AI companies are constantly improving and extending the capabilities of these development tools.
“The more diverse and unbiased data we have, the more robust and safer the algorithms that make up AI systems like deep neural networks become,” says Danny Shapiro, vice president of automotive at NVIDIA. “We also define key performance metrics to measure the collected data quality and add synthetic data into our training datasets. The ultimate goal is to continuously add training data to build a comprehensive matrix of locations, conditions and scenarios.”
The constant enrichment and refinement of algorithm training data is essential to maintain momentum of AV technology development and advancement. It also sets the stage for more sophisticated simulation, one of the primary movers of AV design innovations.
“Self-driving technology must be evaluated again and again during development in a vast array of driving conditions to ensure that the vehicles are far safer than human-driven vehicles,” says Shapiro. “Simulation runs test-drive scenarios in a virtual world, providing rendered sensor data to the driving stack and carrying out driving commands. Re-simulation plays back previously recorded sensor data to the driving stack. The AI model is finally validated against a large and growing collection of test data.”
Taking AV Simulation to the Next Level
Algorithm training data extracted from sensory and operational sources has taken AV developers only so far. It is not enough to provide for all variables encountered when operating driverless vehicles.
The problem is that it’s impossible for current AV test fleets to encounter all of the rare and dangerous scenarios and conditions necessary to train a self-driving car to safely handle the real world.
For example, a small child wearing dark clothing running out in the street at night is not a common scenario that can be repeatedly tested, but it’s one that a self-driving car must be able to safely and properly react to. Current physical vehicle testing can’t validate at a large enough scale. It is limited to fleet size and the geography of the testing area.
Recent advances in AI and computing technologies, however, now make it possible for simulation to fill in the gaps by safely testing and validating self-driving designs and technology before it is deployed.
“High fidelity, time-accurate simulation platforms, such as NVIDIA DRIVE Sim, provide a physically accurate digital twin of driving environments to enable the development and validation of AVs at scale, without putting others on the road in harm’s way,” says Shapiro. “This allows for greater efficiency, cost-effectiveness and most importantly safety, when compared with real-world test drives.”
The ability to simulate the driving environment, traffic and vehicle behavior with dedicated models for each domain enables AV tests to play out unscripted. Automotive designers can use simulation to test edge cases and mine for the unknown variables that a vehicle could encounter in the real world.
Once discovered, these simulated scenarios can be used to test self-driving vehicles iteratively, improving the car’s hardware and software before deployment. With this level of simulation, autonomous vehicle manufacturers can accelerate development and reduce valuable time and cost.
The advanced technology, however, presents a whole new set of challenges. Reconstructing real-world driving scenarios and generating realistic data through simulation can be time-consuming and labor intensive, requiring skilled engineers. Even then, it can be difficult.
Synthesizing Real-World Scenarios
To further advance AVs, developers have begun to use two AI-based techniques that enhance simulation by generating realistic synthetic training data and reconstructing real-world driving scenarios.
The first of these techniques is called virtual reconstruction. This method replicates real-world scenarios as fully synthetic 3D scenes. This data can help train algorithms and empower AV simulations.
Developers begin by converting a digital twin of the road network in question into a drivable simulation environment, which the engineers then use to recreate events, such as navigating a construction zone. If any gaps occur, the developers apply an AI-based traffic model to the tracked object to predict how it would react and fill in the gaps in its trajectory.
Virtual reconstruction helps developers to find potentially challenging situations to train and validate the AV system, using high-fidelity, sensor-generated data to enhance AI behavior models that the engineer can use to create new scenarios. Data from the scenarios can also train the behavior model.
The second method, called neural reconstruction, uses AI to turn recorded video data into simulation. The process begins with recorded driving data. AI identifies and extracts key dynamic elements from this material and loads them into high-fidelity simulations that can be changed as engineers see fit. This approach allows closed-loop testing and full interaction between the AV software and the world it’s driving in.
A Look at AI Under the Hood
AI has not only redefined the way AVs are designed, but it also plays critical roles in areas such as driverless vehicle control and safety (Fig. 2).
“AI and [machine learning] enable vehicle control systems to assess risk and make decisions to prevent accidents,” says Sarika Gupta, chief technology officer at CerebrumX. “Vehicles equipped with AI technologies can analyze data from various sensors—such as cameras, lidar and radar—and identify potential hazards on the road. AI algorithms can then make decisions to avoid those hazards, such as slowing down or changing lanes. This technology has the potential to greatly reduce the number of accidents.”
While risk assessment holds great potential, the technology is also one of the AV’s greatest vulnerabilities.
“Even the most advanced systems available today cannot be relied upon to provide sufficient warning in case of risky scenarios, and they therefore require a human to continuously monitor the situation and be ready to take over immediately,” says Nauto’s Alpert.
Developers hope that as AI-enhanced simulation matures and delivers greater accuracy and visibility, control algorithms could deliver safer and more reliable decision-making and control.
Have AVs Found Their Voice?
Natural language processing advancements are making their way into next-generation vehicles. These systems can often handle sophisticated queries and allow the driver or passengers to communicate with in-car systems naturally.
But the matchup of AI technology with in-car voice applications isn’t cut and dry. AV developers today must decide which flavor of AI voice is most appropriate for the driverless car.
One option is conversational AI. Good examples of this strain of the technology can be found among today’s digital assistants. This is a more mature technology, and as a result, it has already begun to carve out its niche.
“From a technology readiness perspective, in-car voice assistance technologies such as Amazon’s Alexa, Apple’s Siri and Google’s Assistant are already deployed in many vehicles across the globe,” says Duncan Curtis, senior vice president of product at Sama. “Those systems have been well designed for the in-car experience.”
Another option, generative AI, such as ChatGPT, is still in its early days.
This flavor of AI focuses on developing unsupervised and semisupervised algorithms, and it is capable of producing new content, such as text, audio, video, images and code, from existing data.
“Generative AI has the potential to enable a host of new applications,” says Alpert. “This includes dramatically improving what an in-car voice assistant can do. For example, it is possible to use voice recognition to ask a navigation app to find the nearest coffee shop. A more advanced assistant based on generative AI and large language models will be capable of answering more complex, personalized questions, such as ‘find me a gas station with clean restrooms and cheap gas that does not add more than a 5-minute detour.’ Such an assistant will be better at understanding the driver’s intent and provide richer responses.”
Issues, however, continue to compromise generative AI’s reliability, and these flaws are hindering adoption.
“As for ChatGPT and other generative AI type systems, none have been deployed at scale at this stage,” says Curtis. “We are still in the early days of understanding how to enable something like ChatGPT within a vehicle in a safe manner. It would be easy, for example, to give it access to music functions, but core vehicle driving and safety functionality is a long way away.”
A third option that has been introduced recently, called SoundHound Chat AI for Automotive, offers an in-vehicle voice assistant that combines generative AI capabilities combined with a conversational AI-based voice assistant.
This platform promises to give drivers and their passengers access to a large pool of information domains (Fig. 3).
AI technology developers contend that their system can respond to compound queries from users, such as “roll down the windows and turn off the air conditioning,” as well as complex queries that pull knowledge from a variety of domains, such as “turn on air conditioning, and navigate to the nearest salon, where I can get a manicure, and that is open past 6 p.m.”
Fig. 3: Conversational AI can give autonomous vehicles and passengers access to multi-domain information in real time and provide voice-enabled in-car controls. Image courtesy of SoundHound.
“By going beyond command-and-control technology, and anticipating driver needs, advanced voice AI allows automakers to leave step-by-step voice menus behind and deliver conversational, convenient and faster voice experiences,” says Matt Anderson, director of business development for automotive at SoundHound. “In simplifying the overall design of the vehicle’s [user experience], modern AI-driven voice technology also reduces the number of hard and soft buttons, and improves the overall safety of the system while freeing up valuable space for the manufacturer.”
Given the options available and the mix of capabilities, it is a good bet that AV developers will take a while before they settle on a standard AI approach.
More NVIDIA Coverage
Subscribe to our FREE magazine,
FREE email newsletters or both!Latest News
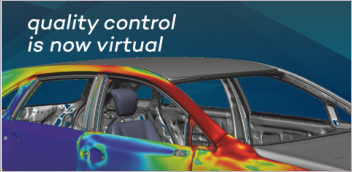
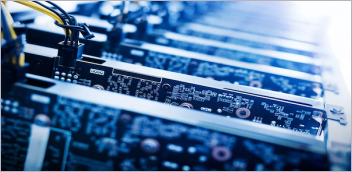
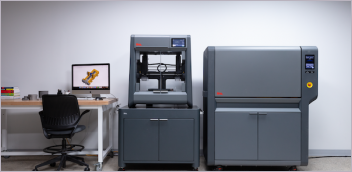
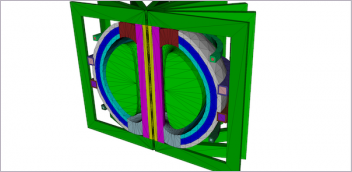